Deep learning, a subset of artificial intelligence inspired by the human brain, has revolutionised various domains by learning from vast amounts of data.
These breakthroughs have showcased the potential of deep learning in pushing the boundaries of what machines can achieve in terms of understanding images, interpreting text, and generating human-like responses. By emulating the intricate connections and information processing of the human brain, deep learning has paved the way for significant advancements in artificial intelligence and has become a cornerstone technology in the quest for intelligent systems.
Key Takeaways
- Deep learning has revolutionised AI by enabling machines to understand images, interpret text, and generate human-like responses.
- The evolution of deep learning has been marked by historical milestones and key contributions from researchers.
- Deep learning architectures such as CNNs, RNNs, and GANs have transformed computer vision and natural language processing.
- Applications of deep learning extend to various fields, including autonomous systems like self-driving cars and smart home devices.
- Despite its advancements, deep learning faces challenges such as ethical considerations and scalability issues, which need to be addressed for future progress.
The Evolution of Deep Learning in AI
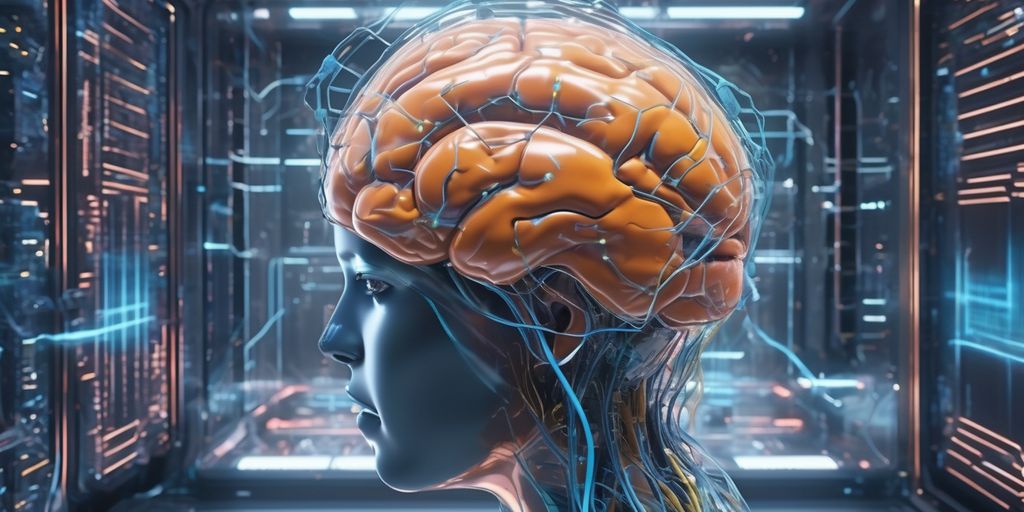
Historical Milestones in Deep Learning
Deep learning has emerged as a powerful technique within machine learning, enabling AI systems to learn and make decisions by simulating the neural networks of the human brain. This approach has revolutionised fields such as computer vision and natural language processing. The history and evolution of machine learning dates from the early esoteric beginnings of neural networks to recent breakthroughs in generative AI.
Key Contributors to Deep Learning Research
Several key contributors have played pivotal roles in advancing deep learning research. Their work has laid the foundation for many of the modern applications we see today. Some notable contributors include:
- Geoffrey Hinton
- Yann LeCun
- Yoshua Bengio
These researchers have significantly influenced the development of deep learning architectures and techniques.
Impact of AI Winter on Deep Learning
The AI Winter had a profound impact on the development of deep learning. During this period, funding and interest in AI research dwindled, slowing progress. However, the resurgence of AI with machine learning techniques has led to remarkable progress. The introduction of deep learning has revolutionised computer vision and natural language processing and holds immense potential to drive further innovations in various fields.
At the core, you’ll find deep learning—an advanced feature of ML whose algorithm has its own learning mechanisms. As the heart of AI, the future of deep learning is closely related to the machine learning industry.
Deep Learning Architectures and Techniques
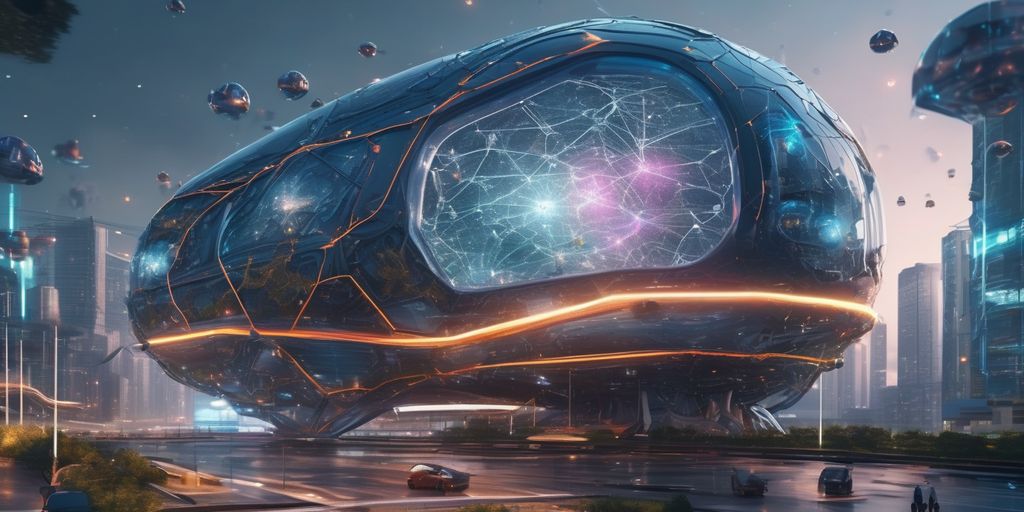
Deep Learning has become the centre of AI research, enabling machines to recognise patterns, learn from data, and make decisions. The recent breakthroughs in deep learning architectures have allowed computers to extract complex features from vast amounts of data.
Convolutional Neural Networks (CNNs)
Convolutional Neural Networks (CNNs) have revolutionised the field of computer vision. These networks are designed to automatically and adaptively learn spatial hierarchies of features from input images. CNNs are particularly effective for image recognition tasks, where they have achieved state-of-the-art results.
Recurrent Neural Networks (RNNs)
Recurrent Neural Networks (RNNs) are a class of neural networks that excel in processing sequential data. They are widely used in applications such as language modelling and time-series prediction. RNNs maintain a memory of previous inputs, making them suitable for tasks where context is crucial.
Generative Adversarial Networks (GANs)
Generative Adversarial Networks (GANs) consist of two neural networks, a generator and a discriminator, that compete against each other. This architecture has been groundbreaking in generating realistic images, videos, and even audio. GANs have opened new avenues in creative AI applications, from art generation to data augmentation.
The rise of Deep Learning has transformed AI from sci-fi to reality, impacting industries with machine learning, neural networks, NLP, and robotics for a transformative future.
Applications of Deep Learning in Computer Vision
Deep learning has propelled significant advancements in computer vision. These advancements have found practical applications in critical areas such as autonomous vehicles, medical imaging, and security systems. Deep learning models excel in tasks such as object recognition, image segmentation, and the generation of realistic images.
Advancements in Natural Language Processing with Deep Learning
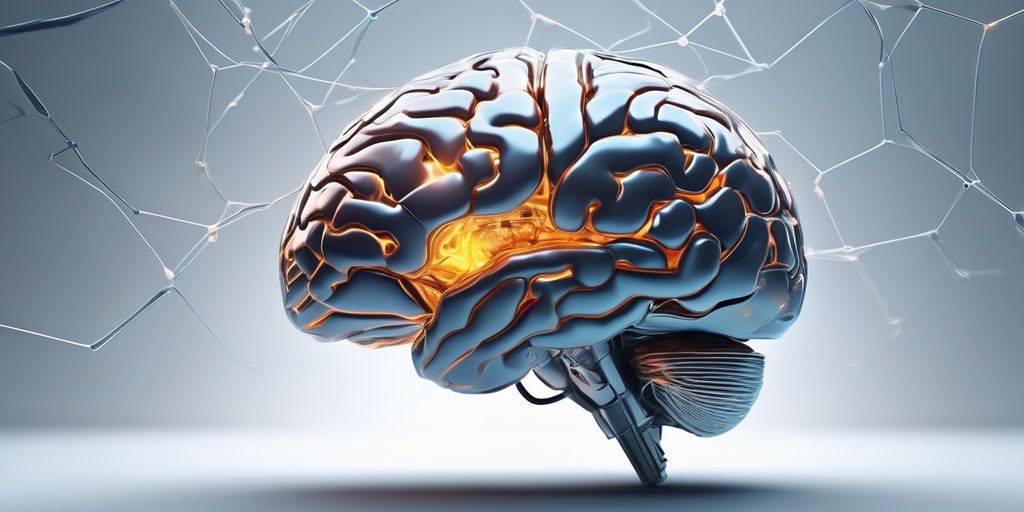
Deep learning has revolutionised natural language processing (NLP), enabling machines to comprehend, generate, and respond to human language. These advancements have significantly improved various applications, making interactions with digital systems more intuitive and human-like.
Sentiment Analysis
Deep learning models have enhanced sentiment analysis by accurately identifying and interpreting emotions in text. This has been particularly beneficial for businesses in understanding customer feedback and improving their services.
Machine Translation
Machine translation has seen remarkable progress with the integration of deep learning techniques. Neural networks can now translate languages with higher accuracy and fluency, bridging communication gaps across the globe.
Text Generation
Text generation capabilities have been greatly improved through deep learning. These models can now produce coherent and contextually relevant text, aiding in content creation and automated responses.
Exploring the eighth generation of AI: breakthroughs in ML algorithms, NLP advancements, integration with IoT and edge computing. Challenges include bias, data privacy, and ethical considerations.
Challenges and Future Directions in Deep Learning
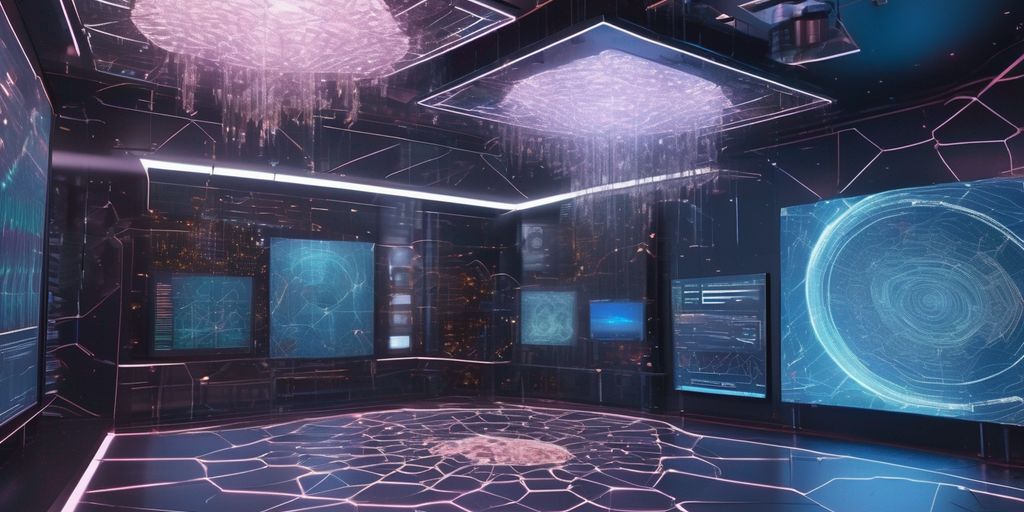
Ethical Considerations
The rise of deep learning has brought about significant ethical concerns. Issues such as bias in algorithms, data privacy, and the potential misuse of AI technologies are at the forefront. Ensuring fairness and transparency in AI systems is crucial to gaining public trust. Researchers are actively working on methodologies to mitigate these ethical challenges.
Scalability Issues
Deep learning models often require vast amounts of data and computational power. This poses a challenge for scalability, especially for smaller organisations. Techniques such as model compression and federated learning are being explored to address these issues. The future of machine learning will likely see more efficient algorithms that can operate with limited resources.
Future Research Opportunities
The field of deep learning is ripe with opportunities for future research. Key areas include improving the explainability and interpretability of models, developing more robust algorithms, and exploring new architectures. Today's problems trigger tomorrow's solutions, and ongoing research is essential for the continued advancement of deep learning technologies.
The future of deep learning holds immense potential, but it is not without its challenges. Addressing these issues will be key to unlocking the full capabilities of AI systems.
The Role of Deep Learning in Autonomous Systems
Self-Driving Cars
Self-driving cars are one of the most prominent applications of deep learning in autonomous systems. Reinforcement learning algorithms process multiple inputs of sensory data to make real-time decisions during navigation. These algorithms enable vehicles to adapt to various driving conditions, ensuring safety and efficiency on the roads.
Drones and Robotics
In the realm of drones and robotics, deep learning has enabled significant advancements. Autonomous robots can now adapt to new tasks through interaction, learning how to manipulate objects or navigate environments independently. This adaptability is crucial for applications ranging from industrial automation to exploration.
Smart Home Devices
Deep learning also plays a vital role in the development of smart home devices. These devices leverage deep learning algorithms to understand and predict user behaviour, enhancing the overall user experience. From voice-activated assistants to intelligent security systems, the integration of deep learning in smart home technology is transforming how we interact with our living spaces.
The integration of deep learning in autonomous systems is not just a technological advancement; it represents a significant step towards creating more intelligent and responsive environments.
Deep learning is revolutionising the field of autonomous systems, enabling machines to perform complex tasks with unprecedented accuracy and efficiency. From self-driving cars to advanced robotics, the applications are vast and transformative. To stay updated on the latest advancements in AI and deep learning, visit our website and explore our comprehensive resources.
Conclusion
In conclusion, the advancements in artificial intelligence, particularly in machine learning and deep learning, have significantly transformed the technological landscape. These breakthroughs have demonstrated the immense potential of deep learning in pushing the boundaries of machine capabilities, from understanding images and interpreting text to generating human-like responses. By emulating the intricate connections and information processing of the human brain, deep learning has become a cornerstone technology in the quest for intelligent systems. As researchers continue to explore and innovate, the future of AI holds promising possibilities for further advancements, driving innovation across various fields and enhancing our ability to solve complex problems.
Frequently Asked Questions
What is deep learning and how does it differ from traditional machine learning?
Deep learning is a subset of machine learning that uses neural networks with many layers (hence 'deep') to analyse various types of data. Unlike traditional machine learning, which often requires manual feature extraction, deep learning automatically learns to identify features from raw data.
How has deep learning impacted the field of artificial intelligence?
Deep learning has significantly advanced the field of AI by enabling machines to perform complex tasks such as image and speech recognition, natural language processing, and autonomous driving. It has become a cornerstone technology in the quest for intelligent systems.
What are some common applications of deep learning?
Common applications of deep learning include image recognition, object detection, machine translation, sentiment analysis, and text generation. These applications span across various industries, from healthcare to automotive.
What challenges does deep learning currently face?
Some of the challenges in deep learning include ethical considerations, such as bias and privacy concerns, scalability issues related to the computational resources required, and the need for large amounts of labelled data for training models.
Who are some key contributors to the field of deep learning?
Key contributors to deep learning include researchers like Geoffrey Hinton, Yann LeCun, and Yoshua Bengio. Their pioneering work in neural networks and deep learning algorithms has laid the foundation for many of the advancements we see today.
What is the future of deep learning?
The future of deep learning holds immense potential, with ongoing research focusing on improving model efficiency, addressing ethical concerns, and exploring new applications. Future directions may include more advanced autonomous systems, enhanced natural language understanding, and breakthroughs in healthcare and personalised medicine.