Artificial Intelligence (AI) is changing our world, but it's not perfect.
One big problem is bias. Bias in AI doesn't just come from nowhere; it often reflects the unfairness in our society. This bias can make things worse for already marginalised groups and can lead to unfair decisions. Fixing this problem isn't just about technology; it's about doing what's right. We need to make sure AI is fair and trustworthy for everyone.
Key Takeaways
- AI bias mirrors societal unfairness and can make existing problems worse.
- Marginalised groups are often the most affected by biased AI systems.
- Trust in AI technologies is hurt when bias is present, making people less likely to use them.
- Detecting and fixing bias in AI is both a technical and moral challenge.
- Making AI fairer can help build a more just and equal society.
Understanding the Origins of Bias in AI
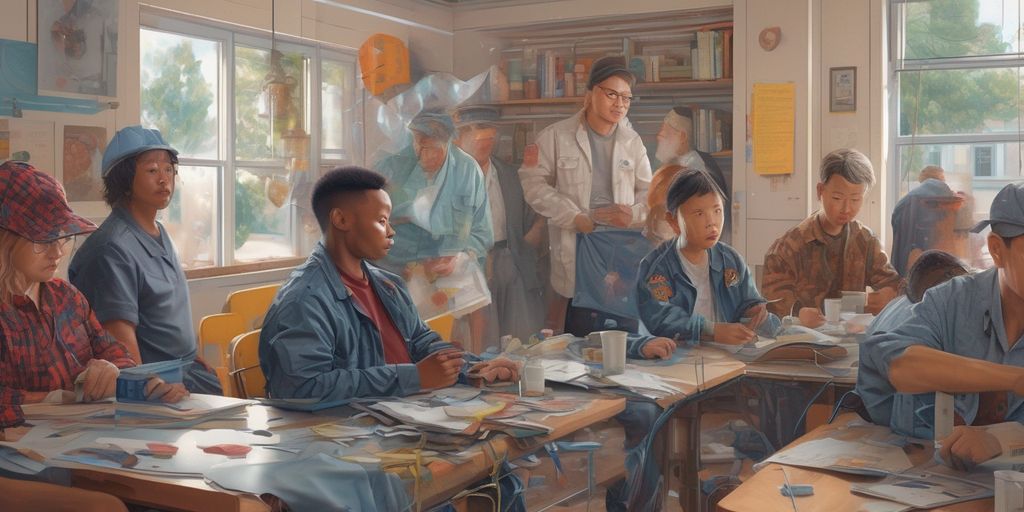
Artificial intelligence (AI) bias happens when AI systems unintentionally reflect prejudices from their training data or design. This bias can be broadly categorised into three types: algorithmic bias, data bias, and interpretation bias. Each type has its own origins and impacts on AI systems.
Historical Data and Systemic Inequalities
AI often mirrors societal issues, with biases in historical data reflecting systemic inequalities. When AI systems are trained on historical data, they can inherit and perpetuate these biases, leading to unfair outcomes. For example, if a dataset used to train an AI system contains biased information, the AI will likely produce biased results.
Bias in Algorithm Design
Algorithmic bias occurs when the algorithms themselves are flawed. This can happen due to the way algorithms are designed or the assumptions made during their development. If the design process does not account for potential biases, the resulting AI system can produce biased outcomes. It's crucial to incorporate ethical considerations and fairness into the design process to mitigate this risk.
The Role of Human Prejudices
Human prejudices play a significant role in the development of AI systems. The individuals who design and train these systems may unintentionally introduce their own biases into the AI. This can happen through the selection of training data, the design of algorithms, or the interpretation of results. Addressing human prejudices is essential to creating fair and unbiased AI systems.
Tackling AI bias is not just a technical challenge but a moral imperative to ensure fairness, equity, and trust in AI applications.
The Impact of AI Bias on Society
Marginalised Groups and Discrimination
AI systems are being used to take decisions. However, there is the risk that such decisions are biased, either because of flaws in the data used to build the systems or because of the way the algorithms are designed. Bias in AI can reinforce stereotypes and social divides, disproportionately affecting marginalised groups. This can lead to discrimination in areas like hiring, lending, and law enforcement.
Trust and Adoption of AI Technologies
Bias in AI undermines trust and hampers the adoption of AI technologies. When people see that AI systems can be unfair, they are less likely to trust and use them. This lack of trust can slow down the adoption of AI in various sectors, from healthcare to finance, where these technologies could otherwise bring significant benefits.
Quality and Fairness in Decision-Making
The quality and fairness of decision-making are crucial for any system, and AI is no exception. When AI systems are biased, the decisions they make can be unfair and of poor quality. This not only affects individuals but can also have broader societal impacts, such as reinforcing existing inequalities and creating new ones.
Addressing AI bias is not just a technical challenge but a moral imperative. Ensuring fairness, equity, and trust in AI applications is essential for a stable and just society.
Identifying Bias in AI Systems
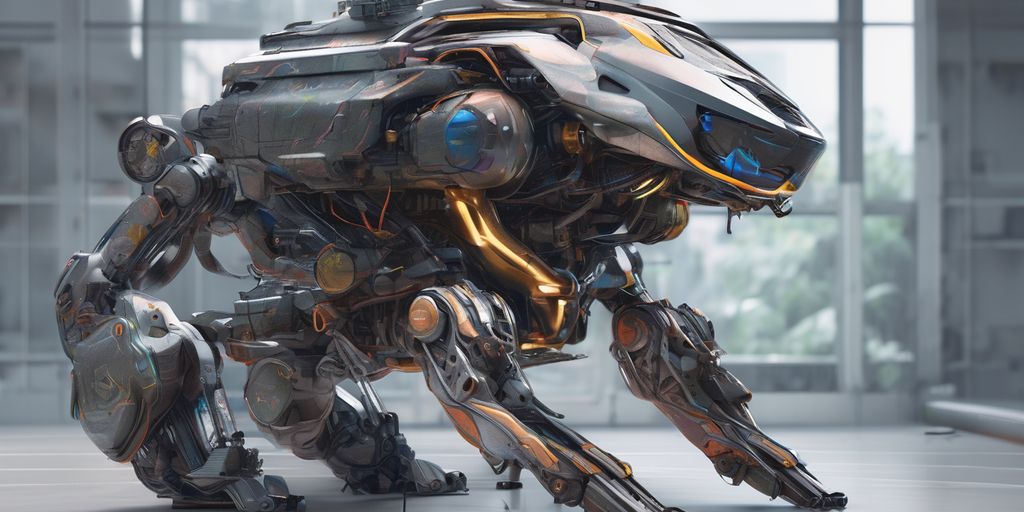
Methods to Detect Bias
Detecting bias in AI systems is a crucial step toward ensuring fairness and effectiveness. This process involves critically examining datasets, algorithms, and their outputs using sophisticated tools and metrics. Engineers have designed AI systems that can spot bias in real-world scenarios. AI could be designed to detect bias within other AI systems or within itself.
Challenges in Measuring AI Bias
However, the task is often challenging due to the complexity and lack of transparency in many AI models. Bias in AI often mirrors societal issues, with biases in historical data reflecting systemic inequalities. By bringing principled thinking to the data and functionality of our systems, we can mitigate, or better, eliminate AI bias risks, and advance a more equitable and stable society along the way.
Case Studies of Biassed AI
Let's explore how bias can be identified in three industries: healthcare, finance, and customer support. In healthcare, AI systems have shown biases in diagnosing diseases, often underdiagnosing conditions in marginalised groups. In finance, biased algorithms have led to unfair loan approvals, disproportionately affecting certain demographics. In customer support, AI chatbots have exhibited biased responses based on the user's language or accent.
Understanding AI bias & fairness is the first step in mitigating AI bias. When considering bias, typically we consider two kinds: bias in the data and bias in the algorithm.
Mitigating Bias in AI
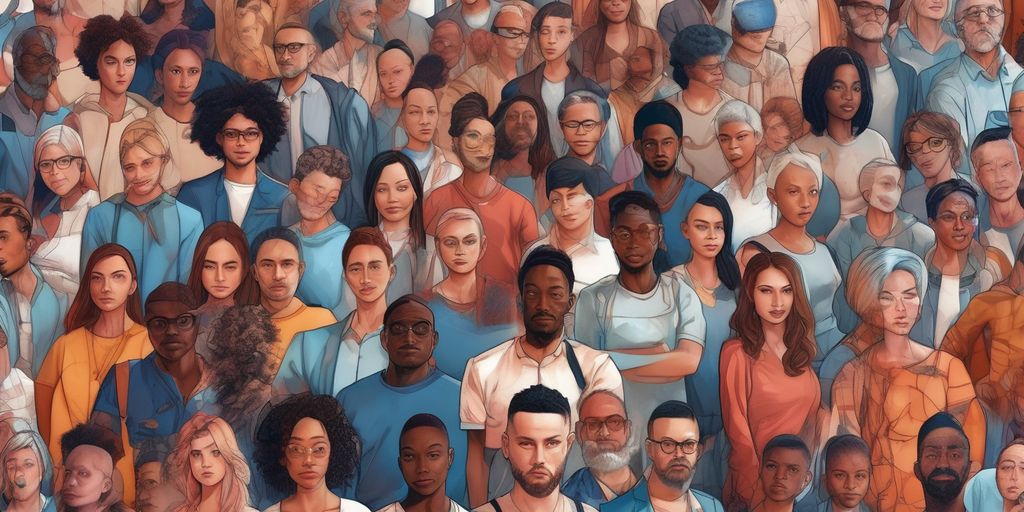
Technical Solutions to Reduce Bias
One primary strategy to mitigate bias in AI algorithms is to ensure diverse and representative data. AI systems learn from data, and biased data leads to biased outcomes. Regularly updating datasets to reflect current realities can help in reducing bias. Additionally, implementing "human-in-the-loop" processes allows for continuous monitoring and adjustment of AI systems.
Ethical Considerations and Fairness
Addressing bias in AI is not just a technical challenge but a moral imperative. Ensuring fairness and equity in AI applications is crucial for maintaining public trust. This involves setting up ethics guidelines and standards that developers must follow. Regular audits and transparency in AI decision-making processes can also help in identifying and mitigating bias.
Principled Approaches to Data Handling
Proper data handling is essential in mitigating bias. This includes collecting data from diverse sources and ensuring it is representative of the population. Continuous monitoring and updating of data are necessary to keep AI systems fair and unbiased. Moreover, involving a diverse team in the development process can provide different perspectives and help in spotting potential biases early on.
AI as a Reflection of Societal Issues
Mirroring Social Inequalities
AI systems often reflect the inequalities present in society. These technologies, much like literature and art, serve as mirrors showing the norms and biases of the societies that created them. When AI uses historical data, it can unintentionally continue systemic inequalities, making it crucial to address these issues at the data level.
Amplifying Historical Biases
AI can also amplify historical biases. If the data used to train AI includes biased information, the AI will likely produce biased outcomes. This can lead to unfair treatment of certain groups, reinforcing existing prejudices. It's essential to bring principled thinking to the data and functionality of our systems to mitigate these risks.
The Role of Language in AI Bias
Language plays a significant role in AI bias. The words and phrases used in training data can carry hidden biases, which the AI then learns and replicates. This can affect everything from search engine results to automated customer service responses. Addressing language bias is a key step in creating fairer AI systems.
Bias in AI in the Workplace
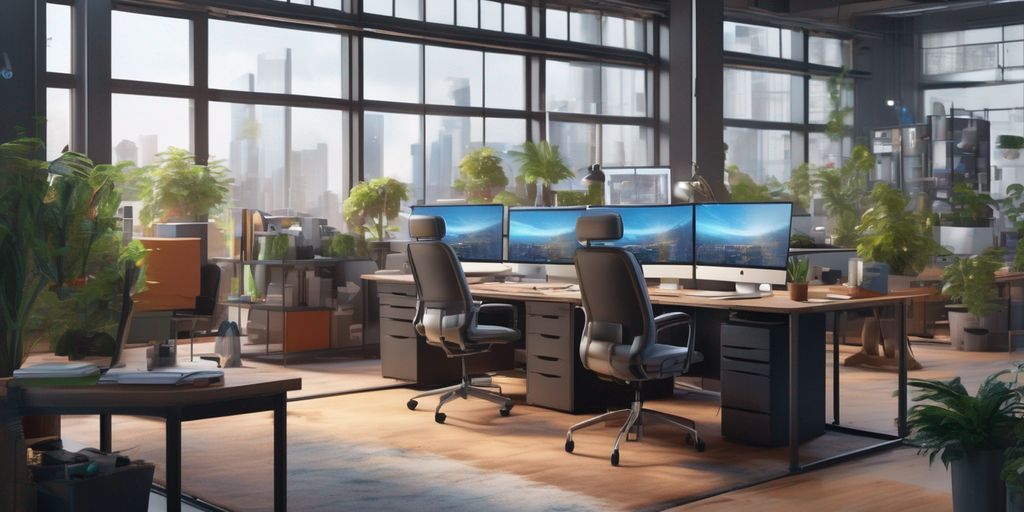
Representation in AI-Generated Images
One way bias in AI shows up is through the images AI systems create when asked certain questions. Much like traditional media, AI-generated images often show scientists, doctors, and engineers mostly as white and male. This issue is hard to fix, making it tough to change these biased views.
Challenges in Retraining AI Systems
Retraining AI systems to remove bias is not easy. These systems learn from past data, which often includes old prejudices. If a company has a history of hiring more men, the AI will think men are better for the job. This makes it hard to change the AI's mind.
Impact on Employment and Opportunities
Bias in AI can affect job chances and career growth. It can make unfair choices, like picking one group over another. This can hurt trust in AI and make people less likely to use these technologies.
Addressing bias in AI is not just a technical challenge but a moral duty to ensure fairness and trust in AI applications.
Artificial Intelligence (AI) can be biased, and this can affect the workplace. It can lead to unfair treatment of employees. To learn more about how AI bias impacts jobs and what can be done to fix it, visit our website.
Conclusion
Bias in AI is a serious issue that affects trust and fairness in technology. It often mirrors the inequalities in our society, making it a moral duty to address. By understanding and fixing these biases, we can create fairer and more reliable AI systems. This not only improves technology but also helps build a more just and equal society. Tackling AI bias is not just about better machines; it's about a better future for everyone.
Frequently Asked Questions
What is AI bias?
AI bias happens when AI systems show unfair preferences or prejudices. This can come from the data they are trained on or the way they are designed.
How does AI bias affect society?
AI bias can harm society by making unfair decisions. It can hurt marginalised groups, reduce trust in AI, and make decision-making less fair.
Why is it important to address AI bias?
Fixing AI bias is crucial to ensure fairness and trust in AI. It helps prevent discrimination and promotes equality in decision-making.
How can we identify bias in AI systems?
We can spot AI bias by testing AI systems and looking for unfair patterns. This can involve checking the data and the results the AI produces.
What are some ways to reduce AI bias?
To reduce AI bias, we can use better data, improve AI design, and regularly check AI systems for unfairness. Ethical guidelines and fairness principles are also important.
Does AI only reflect human biases?
Yes, AI often mirrors the biases found in society. Since AI learns from human data, it can pick up and repeat our prejudices and stereotypes.