In an era where Artificial Intelligence (AI) is not just a buzzword but a fundamental driver of innovation, understanding the intricate layers that compose this vast field is essential.
This article delves into the complexities of AI, Machine Learning (ML), Deep Learning, and Neural Networks, unveiling their hierarchical relationship and distinct functionalities. It serves as a primer for people looking to further understand Artificial Intelligence and its transformative potential in 2024.
Key Takeaways
- Machine Learning has evolved significantly over the years, with key milestones marking its progress.
- Understanding the core concepts of Machine Learning, such as algorithms, models, and the role of data, is crucial.
- In 2024, Machine Learning finds applications in diverse fields like healthcare, finance, and retail.
- Ethical considerations, including bias and data privacy, are critical challenges in the field of Machine Learning.
- Numerous tools and technologies, including popular frameworks and cloud-based solutions, are available to support Machine Learning initiatives.
The Evolution of Machine Learning
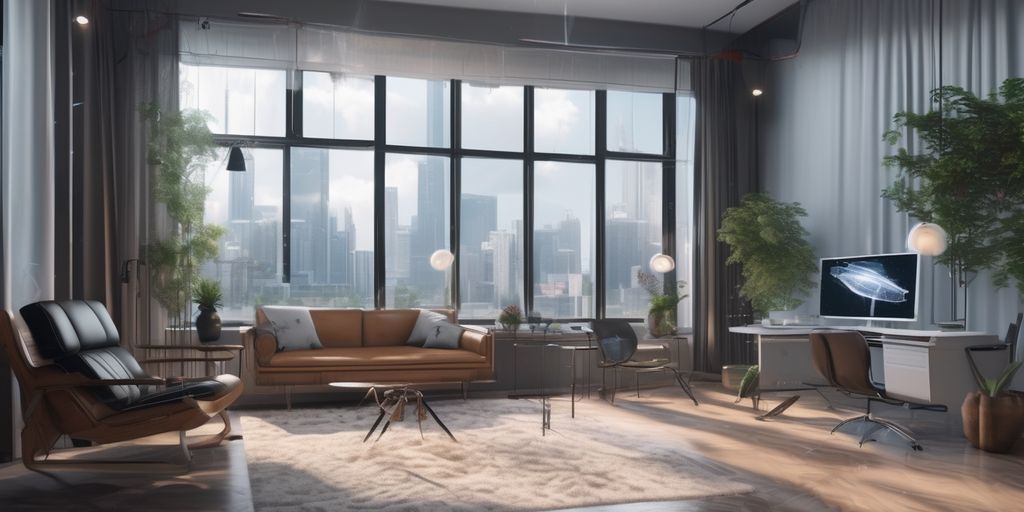
Historical Milestones in Machine Learning
The history and evolution of Machine Learning dates from the early esoteric beginnings of neural networks to recent breakthroughs in generative AI. Initially, symbolic AI dominated the field, using rules and logical reasoning to mimic human intelligence. However, it faced significant challenges until the 1990s, when Machine Learning emerged as a dominant approach. Deep Learning, a subset of Machine Learning, revolutionised AI capabilities in the 2010s.
Key Innovations in Recent Years
In recent years, Machine Learning has seen numerous innovations that have transformed various industries. These include advancements in natural language processing, reinforcement learning, and the development of sophisticated algorithms that can process vast amounts of data. The rise of cloud computing has also played a crucial role, providing the necessary infrastructure for large-scale Machine Learning applications.
Future Trends to Watch
Looking ahead, several trends are poised to shape the future of Machine Learning. These include the integration of Machine Learning with other AI technologies, such as natural language processing and computer vision, as well as the increasing importance of ethical considerations in AI development. Additionally, the continued evolution of quantum computing holds the potential to further accelerate Machine Learning capabilities.
As we move forward, the synergy between Machine Learning and other emerging technologies will likely drive unprecedented advancements, making it an exciting field to watch.
Core Concepts of Machine Learning
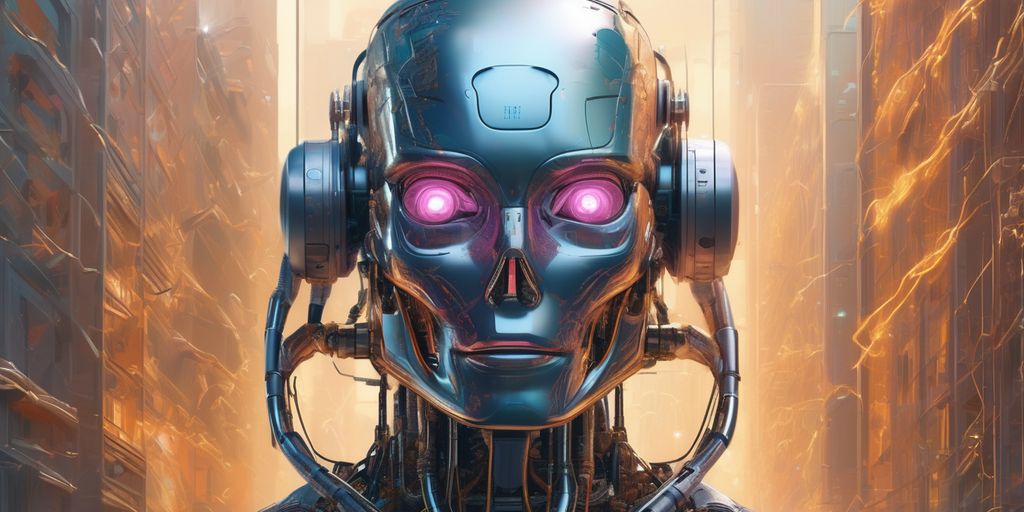
Understanding Algorithms and Models
Machine learning empowers computers to learn from experience, enabling them to perform tasks without being explicitly programmed for each step. Algorithms iteratively learn from data, identifying patterns and making informed decisions. These algorithms can be broadly classified into different types, such as supervised learning, unsupervised learning, and reinforcement learning.
The Role of Data in Machine Learning
Data is the cornerstone of machine learning. The quality and quantity of data directly impact the performance of machine learning models. Data can be structured, like databases, or unstructured, like text and images. The process involves collecting, cleaning, and preparing data to train models effectively.
Supervised vs Unsupervised Learning
In supervised learning, the algorithm is trained on labelled data, where each input is associated with the correct output. The model learns to map inputs to their corresponding outputs and can make predictions on new, unseen data. In contrast, unsupervised learning is used on unlabelled data to discover patterns or groups. This type of learning is often used for clustering and association tasks.
Machine learning and deep learning have revolutionised various domains by emulating the human brain. Deep learning enables understanding images, interpreting text, and generating human-like responses, with applications in autonomous systems.
Applications of Machine Learning in 2024
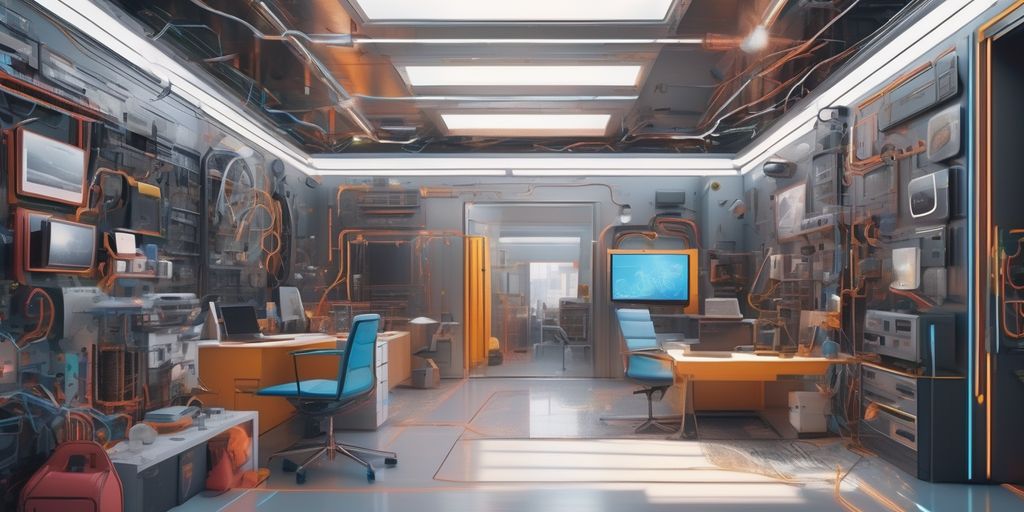
Healthcare and Medicine
In 2024, machine learning continues to revolutionise healthcare by enhancing diagnostic accuracy and personalising treatment plans. Predictive models are used to anticipate disease outbreaks and patient deterioration, enabling timely interventions. From sci-fi to reality, AI-driven tools are now integral in medical imaging, drug discovery, and patient care management.
Finance and Banking
The finance sector leverages machine learning for fraud detection, risk management, and personalised financial services. Algorithms analyse vast datasets to identify suspicious activities and predict market trends. This not only enhances security but also optimises investment strategies and customer experiences.
Retail and Customer Service
Machine learning transforms retail by providing personalised shopping experiences and optimising supply chains. Predictive analytics help retailers manage inventory and forecast demand, while chatbots and recommendation systems improve customer service. The integration of AI in retail operations leads to increased efficiency and customer satisfaction.
The integration of machine learning across various sectors underscores its transformative potential, turning once futuristic concepts into everyday realities.
Challenges and Ethical Considerations
Bias and Fairness in Machine Learning
Machine learning models can sometimes perpetuate or even amplify biases found in their training data, leading to unfair or discriminatory outcomes. Addressing these biases is essential to avoid reinforcing existing societal inequalities. Strategies to mitigate bias include ensuring diverse and representative datasets and implementing fairness-aware algorithms.
Data Privacy Concerns
The use of vast amounts of data in machine learning raises significant privacy concerns. Protecting user data and ensuring compliance with data protection regulations is crucial. Techniques such as data anonymisation and differential privacy can help safeguard personal information while still enabling the benefits of machine learning.
Regulatory and Compliance Issues
As machine learning technologies advance, comprehensive governance and regulations are necessary to ensure ethical deployment and protect user rights. Policymakers and industry stakeholders must collaborate to develop guidelines and standards for responsible AI development. This includes addressing the issue of AI and algorithms, recommending on that matter the creation of a robust regulatory framework.
Ethical AI development must prioritise fairness, transparency, and accountability to build trust and ensure the responsible use of technology.
Tools and Technologies for Machine Learning
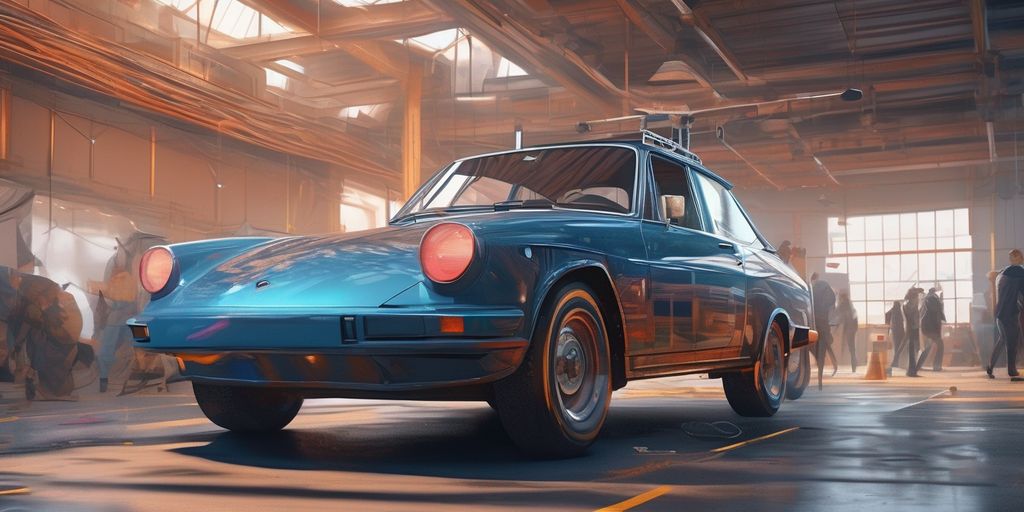
Popular Machine Learning Frameworks
The AI market leaders, including Amazon Web Services, Google Cloud, and Azure, provide robust environments for developing and deploying machine learning models. These platforms offer comprehensive services that span from initial model building to deployment, including features for live data processing and batch output predictions, which cater to a wide range of business needs, from real-time recommendations to data analysis.
Cloud-Based Machine Learning Solutions
Cloud-based solutions have revolutionised the way businesses approach machine learning. These platforms offer scalable resources that can be adjusted based on the workload, making it easier for companies to manage costs and performance. Additionally, they provide tools for collaboration, allowing teams to work together seamlessly regardless of their physical location.
Open Source Tools and Libraries
Open source tools and libraries play a crucial role in the machine learning ecosystem. They offer flexibility and customisation, enabling developers to tailor solutions to specific needs. Some popular open source tools include TensorFlow, PyTorch, and Scikit-learn. These tools are widely used for their extensive documentation and community support.
In a world where technology increasingly shapes our experiences and decisions, understanding machine learning opens doors to unprecedented opportunities. From personalised recommendations that enhance your shopping experience to predictive models that optimise supply chains and improve healthcare outcomes, AI is revolutionising industries and revolutionising how we interact with the world around us.
The Intersection of Machine Learning and Other AI Technologies
Machine Learning vs Deep Learning
Machine Learning (ML) and Deep Learning (DL) are often used interchangeably, but they are distinct fields within the broader spectrum of Artificial Intelligence (AI). Machine Learning focuses on enabling computers to learn from data and make decisions based on that data. In contrast, Deep Learning, a subset of ML, uses neural networks with many layers (hence 'deep') to analyse various factors of data. This distinction is crucial for understanding the capabilities and limitations of each technology.
Integration with Natural Language Processing
Natural Language Processing (NLP) is another critical area of AI that intersects with ML. NLP involves the interaction between computers and humans through natural language. By integrating ML algorithms, NLP systems can improve their ability to understand and generate human language, making applications like chatbots and voice assistants more effective. This integration opens up new possibilities and capabilities in many different types of industries.
The Role of Neural Networks
Neural networks are the backbone of many ML and DL applications. These networks are designed to simulate the way the human brain processes information, allowing machines to recognise patterns and make decisions. The role of neural networks in AI cannot be overstated, as they are fundamental to advancements in image and speech recognition, autonomous driving, and more.
As AI continues to evolve, it is essential to address challenges, prioritise ethical considerations, and responsibly navigate the future of AI.
Getting Started with Machine Learning
Learning Resources and Courses
Embarking on a journey into machine learning can be both exciting and overwhelming. Fortunately, there are numerous resources available to help you get started. Online platforms like Coursera, edX, and Udacity offer comprehensive courses that cover everything from basic concepts to advanced techniques. Additionally, many universities provide free access to their course materials, allowing you to learn at your own pace.
Building Your First Model
Creating your first machine learning model is a significant milestone. Start with a simple dataset and choose a well-documented algorithm. Follow these steps:
- Data Collection: Gather a dataset relevant to your problem.
- Data Preprocessing: Clean and prepare the data for analysis.
- Model Selection: Choose an appropriate algorithm for your task.
- Training: Train the model using your dataset.
- Evaluation: Assess the model's performance using metrics like accuracy or precision.
- Optimisation: Fine-tune the model to improve its performance.
Community and Support Networks
Engaging with the machine learning community can provide invaluable support and insights. Online forums, such as Stack Overflow and Reddit, are excellent places to ask questions and share knowledge. Additionally, joining local meetups or attending conferences can help you connect with other enthusiasts and professionals in the field.
Machine learning is not just a skill; it's a journey of continuous learning and adaptation. Embrace the challenges and celebrate the milestones along the way.
Embarking on your machine learning journey has never been easier. Our comprehensive guides and latest AI news will equip you with the knowledge and tools you need to succeed. Don't miss out on the latest trends and insights in AI. Visit our website today to get started!
Conclusion
As we navigate through 2024, the landscape of Artificial Intelligence and Machine Learning continues to evolve at a rapid pace. By demystifying these concepts, we open the door to a myriad of possibilities where AI is not just a buzzword but a transformative tool that enhances productivity, efficiency, and innovation. Understanding the foundational elements of AI, from Machine Learning to Deep Learning and Neural Networks, equips us with the knowledge to harness its full potential. As we continue to explore and innovate, the importance of staying informed and adaptable cannot be overstated. The journey of demystifying AI is ongoing, and with each step, we move closer to a future where the integration of AI into our daily lives becomes seamless and profoundly impactful.
Frequently Asked Questions
What is the difference between AI, Machine Learning, and Deep Learning?
AI, or Artificial Intelligence, is the broader concept of machines being able to carry out tasks in a way that we would consider 'smart'. Machine Learning is a subset of AI that involves the use of algorithms and statistical models to enable machines to improve at tasks with experience. Deep Learning is a further subset of Machine Learning that uses neural networks with many layers to analyse various factors of data.
Why is it important to demystify machine learning?
Demystifying machine learning is crucial as it allows individuals and organisations to understand and harness the transformative potential of AI in various aspects of life and work. It makes the technology more accessible and less intimidating, encouraging its adoption and innovation.
What are some historical milestones in machine learning?
Some key milestones include the development of the perceptron in the 1950s, the introduction of backpropagation in the 1980s, and the advent of deep learning techniques in the 2010s. Each of these milestones has significantly advanced the field and expanded its applications.
How is machine learning used in healthcare in 2024?
In 2024, machine learning is used in healthcare for predictive analytics, personalised medicine, and diagnostic imaging. It helps in early disease detection, treatment recommendation, and improving patient outcomes by analysing vast amounts of medical data.
What are the ethical considerations in machine learning?
Ethical considerations in machine learning include addressing bias and fairness, ensuring data privacy, and navigating regulatory and compliance issues. It is essential to develop and deploy machine learning models responsibly to avoid perpetuating inequalities and violating privacy rights.
What resources are available for learning machine learning?
There are numerous resources available for learning machine learning, including online courses, tutorials, and textbooks. Platforms like Coursera, edX, and Udacity offer comprehensive courses, while communities and forums provide support and networking opportunities for learners.