In today's world, artificial intelligence (AI) is everywhere.
From smart assistants in our homes to advanced systems in healthcare, AI is changing how we live. But with this power comes a big question: How much data collection is too much? Collecting lots of data can help make AI smarter, but it can also create problems we might not be able to fix. This article looks at the risks, ethics, and ways to handle the challenges of collecting data for AI.
Key Takeaways
- Collecting too much data for AI can lead to serious risks like data poisoning attacks, where bad actors mess with the data to harm the AI system.
- Validating the data collected is crucial to ensure its accuracy and prevent manipulation.
- Balancing innovation with privacy is essential to maintain trust and avoid ethical issues.
- Restricting data sharing and developing strong policies can help protect AI systems from potential threats.
- Transparency in data practises builds trust and helps in maintaining the public's confidence in AI.
The Risks of Excessive Data Collection in AI Systems
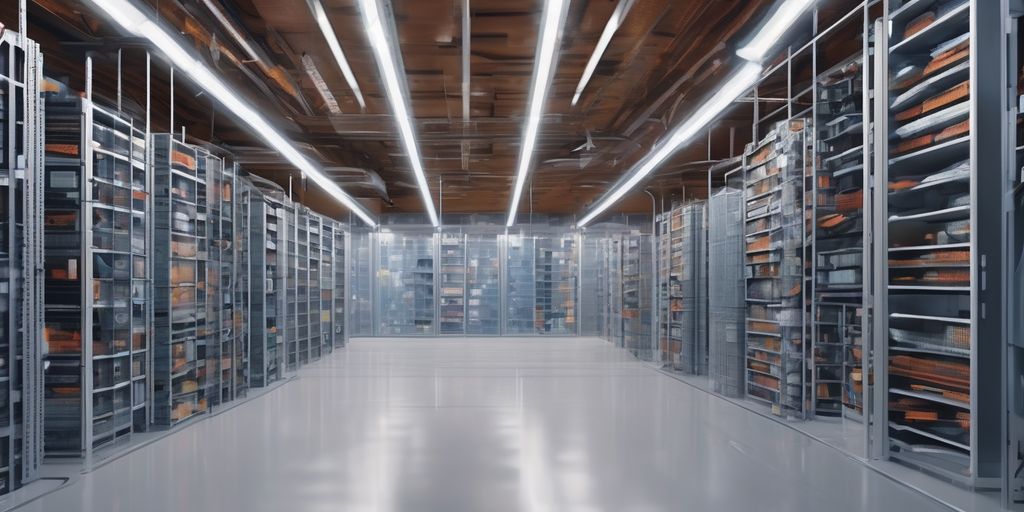
Understanding Data Poisoning Attacks
AI systems are vulnerable to data poisoning attacks, where bad actors intentionally introduce harmful data. This can lead to AI making wrong decisions. AI users must validate their data collection practises to avoid such risks. They should ask themselves questions like: How was the data prepared? Are there inaccuracies? Is any data missing or underrepresented?
The Role of Data Validation
Data validation is crucial in ensuring the quality and reliability of AI systems. By regularly reviewing and securing data collection and sharing policies, AI users can identify and mitigate potential threats. This process should be formal and result in written policies that govern data use.
Implications for AI Security
Excessive data collection can create vulnerabilities that can be exploited. For instance, the process of preparing data for AI training can be time-consuming and may involve non-expert labelers, increasing the risk of data breaches. AI systems can only learn from the data they are trained on, so missing or inaccurate data can lead to malfunctioning systems.
The best and worst of AI in 2024 (so far) show that while AI can be incredibly efficient, it also poses significant privacy concerns and risks of data breaches.
In summary, while collecting large amounts of data can enhance AI capabilities, it also increases the risk of attacks and security issues. Proper data validation and secure practises are essential to mitigate these risks.
Ethical Considerations in AI Data Collection
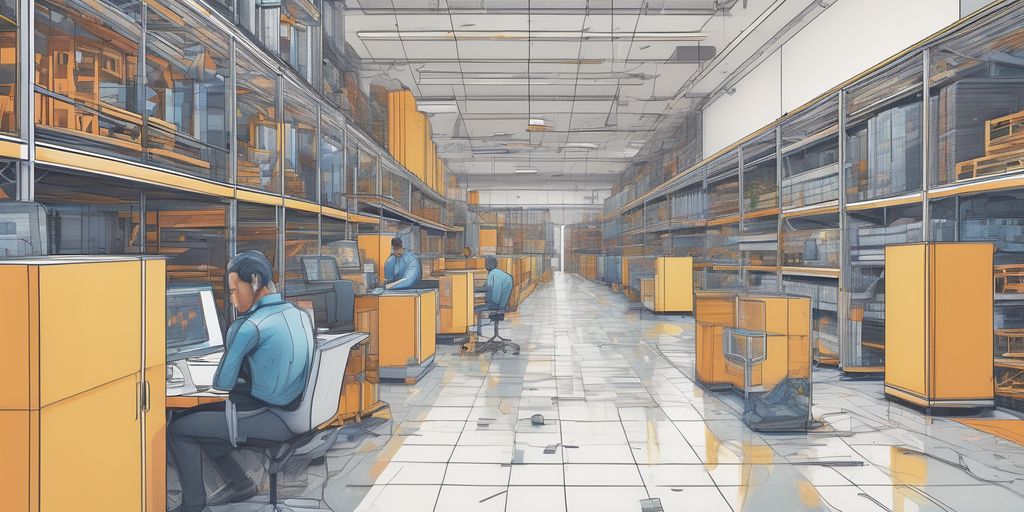
Balancing Innovation and Privacy
In the race to innovate, it's easy to overlook privacy. AI systems need data to learn and improve, but collecting too much can lead to serious privacy issues. Companies must find a balance between gathering enough data to innovate and respecting users' privacy.
The Human Element in AI
AI isn't just about machines; it's about people too. The data collected often includes personal information, making it crucial to consider the human impact. Ethical guidelines should be in place to ensure that data collection respects human dignity and privacy.
Legal and Regulatory Challenges
Navigating the legal landscape is another challenge. Different countries have different laws about data collection and privacy. Companies must stay updated on these laws to avoid legal issues. Formal reviews of data policies can help identify emerging ways data can be weaponized against systems, shaping better practises.
Ethical considerations are not just about following laws but also about doing what's right for people and society.
Strategies to Mitigate AI Data Collection Risks
Implementing Robust Data Validation
To protect AI systems, it's crucial to validate data collection practises. This means checking for manipulated, inaccurate, or incomplete data. By doing so, we can prevent data poisoning attacks that could harm AI systems.
Restricting Data Sharing
AI users must review and secure their data collection and sharing policies. These reviews should be formal and identify new ways data can be weaponised against systems. The outcome should be written policies that govern how data is collected and shared.
Developing Comprehensive Policies
In the age of AI attacks, today's data collection practises may soon be outdated. If an adversary knows an AI user's data collection methods, they can influence the process to attack the AI system. Therefore, it's essential to rethink data practises to protect against such threats.
AI users need to fundamentally rethink their data practises to protect themselves from having it weaponised against them.
The Impact of Data Practises on AI Trustworthiness
Building Trust Through Transparency
Trust in AI systems is built on transparency. When companies openly share their data collection methods, it helps users feel more secure. Clear communication about how data is gathered and used can prevent misunderstandings and build confidence.
The Consequences of Data Manipulation
Manipulating data can have serious consequences. If data is altered or incomplete, AI systems might make wrong decisions. This can lead to a loss of trust. It's important to validate dataset collection practises to avoid these risks.
Public Perception and AI
Public perception of AI is shaped by how data is handled. If people believe their data is safe and used ethically, they are more likely to trust AI systems. On the other hand, if there are concerns about data privacy or misuse, trust can quickly erode.
Future Directions for AI Data Collection
Evolving Data Collection Techniques
AI's energy demands strain global power grids. The rapid growth of AI technology leads to increased energy consumption, impacting power grids and renewable sources globally. New data collection methods must be developed to handle this growth efficiently. These methods should focus on reducing energy use while maintaining data quality.
The Role of Policy in Shaping AI
Policies will play a crucial role in the future of AI data collection. Governments and organisations need to create rules that ensure data is collected ethically and securely. This includes protecting personal information and preventing misuse. Clear guidelines will help build trust and make AI systems more reliable.
Preparing for Emerging Threats
As AI technology advances, new threats will emerge. It's important to stay ahead by identifying potential risks early. Regular reviews of data collection practises can help spot vulnerabilities. By being proactive, we can protect AI systems from attacks and ensure they remain safe and effective.
The future of AI data collection depends on balancing innovation with security. By adopting new techniques and policies, we can harness the power of AI while minimising risks.
ChatGPT and Data Collection: A Case Study
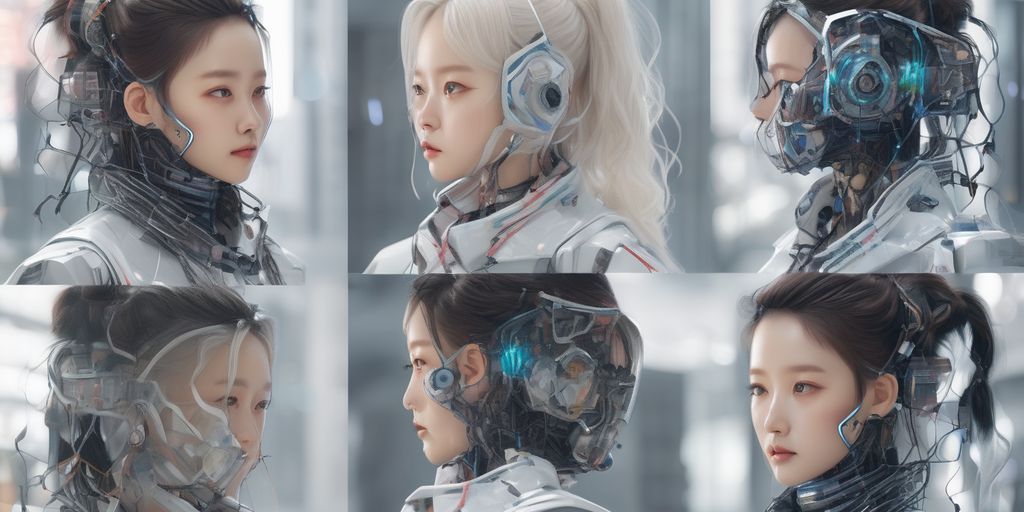
ChatGPT uses a variety of methods to gather data, including user interactions and publicly available information. This data helps improve the model's responses and make it more useful. However, the ability to attack the dataset collection process represents a new challenge. It's important to ensure that the data collected is accurate and not manipulated.
There are several vulnerabilities in the data collection process. For example, it can be difficult to tell if an interaction with the system is a valid use or an attempt to probe for weaknesses. This makes it crucial to validate dataset collection practises to account for risks that manipulated, inaccurate, or incomplete datasets pose to AI systems.
From ChatGPT's experience, it's clear that AI users need to rethink their data practises. They must validate their collection methods and restrict data sharing to protect against potential attacks. These changes will challenge current attitudes towards data but are necessary to ensure the security and reliability of AI systems.
The Balance Between Data Utility and Security in AI
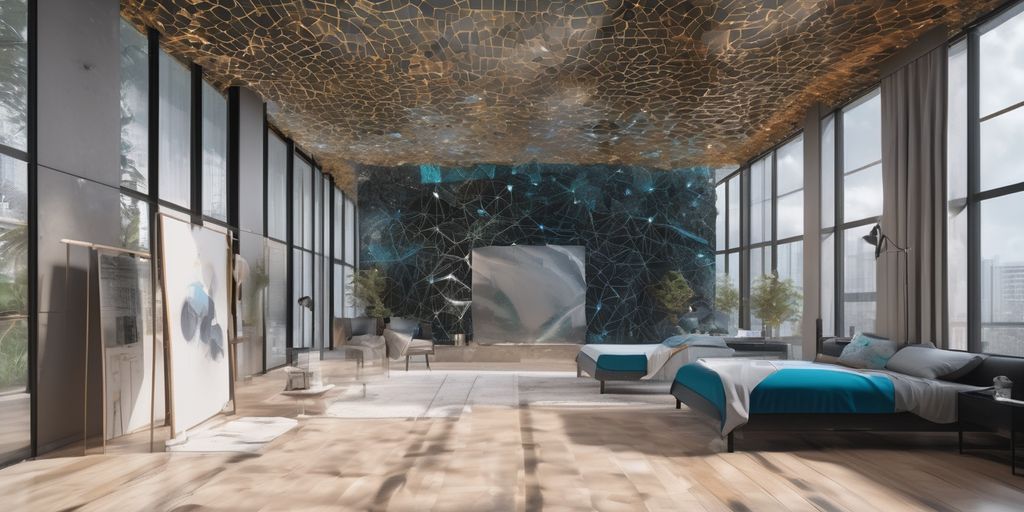
Maximising Data Utility
In the world of AI, data is often seen as "digital gold." The more data an AI system has, the better it can learn and make accurate predictions. However, too much data collection can lead to risks. It's important to find a balance where the AI can still perform well without compromising security.
Ensuring Data Security
Keeping datasets secure is one key to protecting against AI attacks. If adversaries obtain the dataset used, they can manipulate the AI's behaviour. Critical AI systems must restrict how and when the data used to build them is shared. For sensitive applications, data should by default not be shared. Exceptions should be well reasoned and explicitly written.
Finding the Right Balance
Balancing data utility and security is a challenging task. On one hand, we need enough data to make AI systems fair, unbiased, and trustworthy. On the other hand, we must protect this data from being weaponised against the system. Formal reviews of data collection and sharing policies can help identify emerging threats and shape better practises.
In conclusion, the balance between data utility and security in AI is crucial. By implementing robust data validation and restricting data sharing, we can ensure that AI systems remain both effective and secure.
Balancing data utility and security in AI is crucial. While we want AI to be useful, we must also keep our data safe. Curious to learn more? Visit our website for the latest insights and updates on AI.
Conclusion
In the end, the question of how much AI data collection is too much boils down to balance. We must be careful not to gather so much data that it becomes a weapon against us. At the same time, we need enough data to make AI systems smart and useful. It's a tricky line to walk, but it's one we must navigate with care. By being thoughtful about how we collect and use data, we can harness the power of AI without creating a monster we can't control. The future of AI depends on the choices we make today, so let's choose wisely.
Frequently Asked Questions
What are the dangers of collecting too much data for AI?
Collecting too much data can make AI systems vulnerable to attacks, like data poisoning. This is when someone manipulates the data to trick the AI. It can also lead to privacy issues and misuse of personal information.
How can we protect AI systems from data poisoning attacks?
To protect AI systems, it's important to check and validate the data regularly. This means making sure the data is accurate and hasn't been tampered with. Limiting who can access and share the data also helps.
Why is data validation important in AI?
Data validation ensures that the information used by AI is correct and reliable. Without it, AI systems can make wrong decisions based on false or misleading data, which can be harmful.
What ethical issues arise from AI data collection?
AI data collection can invade people's privacy and be used to control or manipulate them. It's important to balance the benefits of AI with respect for people's rights and freedoms.
How can we balance data utility and security in AI?
Balancing data utility and security means using data in a way that benefits AI development while also protecting it from misuse. This can be done by creating strong policies and regularly reviewing data practises.