AI-based predictive analytics is a game-changer that helps forecast future events using data.
This technology can benefit many sectors, but it also has some hurdles. Knowing both the benefits and challenges can help you use this tool better.
Key Takeaways
AI-based predictive analytics can greatly improve decision-making by providing data-driven insights.
This technology boosts efficiency by quickly analysing large amounts of data.
It can help identify risks early, allowing for proactive management.
Personalisation is another advantage, making it easier to tailor services and products to individual needs.
Despite its benefits, there are challenges such as data quality issues and ethical concerns.
Understanding Predictive Analytics
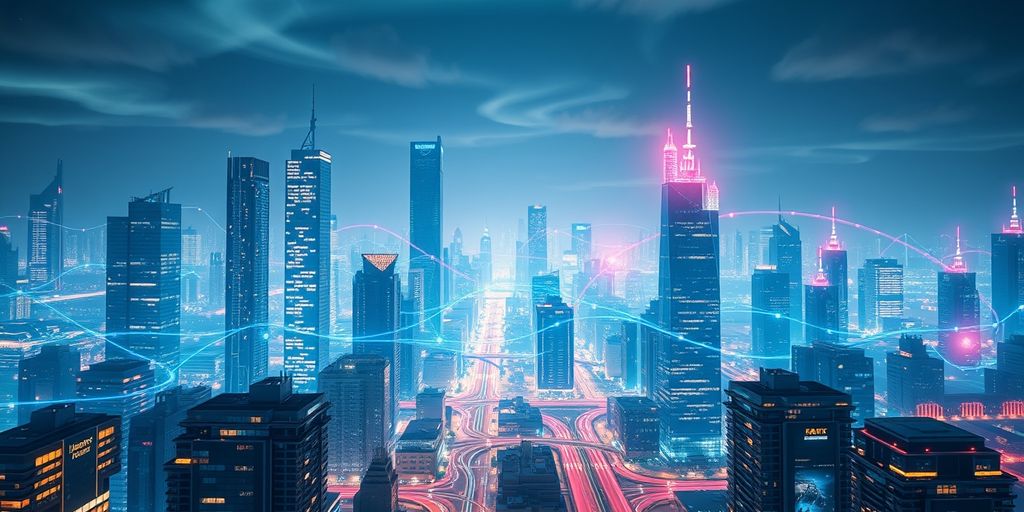
What is Predictive Analytics?
Predictive Analytics is a way to use data to guess what might happen in the future. It looks at old data and uses special tools like machine learning to make these guesses. This helps businesses and other groups to plan better and make smarter choices.
How Does Predictive Analytics Work?
Predictive Analytics involves a sequence of stages, each building upon the last, to convert extensive data sets into forecasts and trends. First, data is collected and cleaned. Then, models are created using algorithms. These models are trained to find patterns in the data. Finally, the models make predictions based on new data.
Key Components of Predictive Analytics
Data Collection: Gathering data from different sources.
Data Cleaning: Making sure the data is correct and ready to use.
Model Building: Creating models using algorithms to find patterns.
Model Training: Teaching the model to understand the data.
Prediction: Using the model to make guesses about future events.
Predictive Analytics can turn huge amounts of data into useful insights, helping organisations to see trends and make better decisions.
Benefits of AI-Based Predictive Analytics
AI-based predictive analytics is a game-changer, offering numerous advantages that can transform how businesses operate and make decisions. Let's dive into some of the key benefits.
Enhanced Decision-Making
With AI-driven insights, you can make more informed decisions, reducing guesswork and improving strategic planning. AI helps you decode complex data to provide actionable insights, making your decision-making process more accurate and reliable.
Increased Efficiency
AI algorithms can analyse vast datasets much faster than human analysts. This leads to increased efficiency in operations, allowing businesses to focus on more critical tasks. Imagine having a tool that can sift through mountains of data in seconds!
Proactive Risk Management
AI predictive analytics can identify potential risks and issues before they escalate. This proactive approach is especially valuable in industries like finance or healthcare, where early detection of risks can save both time and money.
Personalization Opportunities
In sectors like retail or e-commerce, AI predictive analytics helps you personalise offerings. By tailoring products, services, and marketing to individual customer preferences, you can enhance customer satisfaction and loyalty.
The integration of AI in predictive analytics is transforming a wide array of industries. By harnessing the ability to foresee trends and adapt strategies accordingly, organisations across various sectors are gaining significant advantages in their respective, ever-evolving market environments.
Challenges in Implementing Predictive Analytics
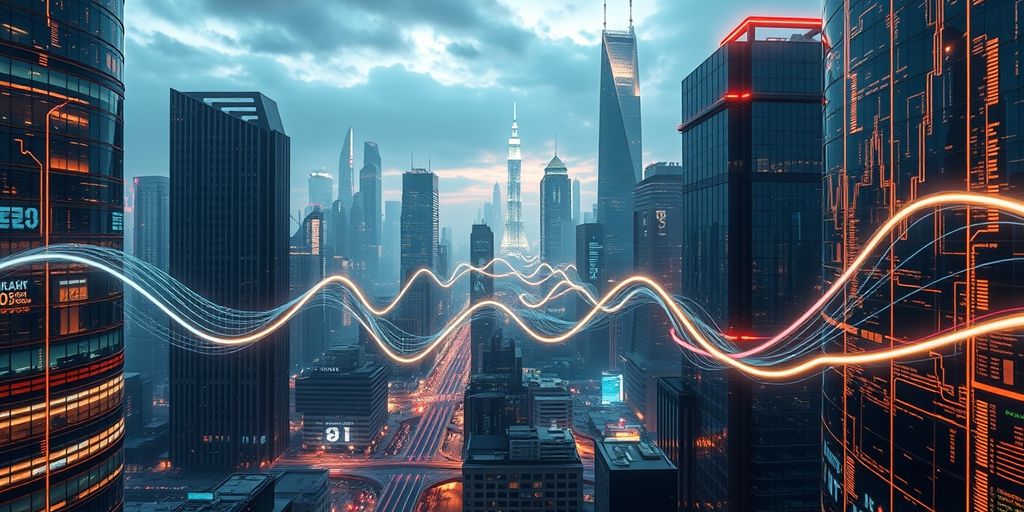
AI-based predictive analytics is a powerful tool that offers a multitude of benefits but also presents certain challenges. Understanding these can help you navigate and leverage this technology more effectively.
Data Quality Issues
The accuracy of AI predictions relies heavily on the quality and quantity of data. Poor-quality data can lead to inaccurate predictions, making it crucial to ensure data is clean and well-organised.
Complexity of Models
Some AI models can be quite complex and hard to understand. This complexity can make it difficult to interpret how the model arrives at certain predictions, which can be a barrier to trust and adoption.
Ethical Considerations
Ensuring that predictive analytics complies with regulations and ethical standards is crucial. Concerns about data ownership and algorithmic bias necessitate transparency and fairness in AI applications.
Navigating these challenges requires a balanced approach, combining technical expertise with ethical considerations to fully harness the power of AI-based predictive analytics.
Applications of Predictive Analytics in Healthcare
Early Disease Detection
Predictive analytics is transforming early disease detection. By analysing vast amounts of patient data, AI models can spot subtle patterns and anomalies that might indicate the onset of diseases, such as cancer or heart conditions, much earlier than traditional methods. This leads to early interventions, which can significantly improve patient outcomes.
Personalised Treatment Plans
AI is instrumental in creating personalised medicine. It considers a plethora of factors like genetic information, lifestyle, and individual health records to tailor treatment plans. This approach not only increases the efficacy of treatments but also minimises the risk of side effects, leading to better patient compliance and overall health outcomes.
Epidemic Outbreak Prediction
AI algorithms can analyse global health data to predict potential epidemic outbreaks, enabling proactive measures. This can help in mobilising resources and planning interventions to curb the spread of diseases.
Diagnostic Imaging
AI enhances diagnostic imaging by providing more accurate interpretations of MRI, CT scans, and X-rays. This leads to better diagnosis and treatment plans, ensuring that patients receive the most effective care possible.
Machine Learning Models for Predictive Analytics
Machine learning has transformed the realm of predictive analytics, giving data analysts the power to create precise models and make well-informed choices. Let's dive into some of the most common machine learning models used in predictive analytics.
Regression Analysis
Regression analysis is a statistical method that helps identify relationships between variables. It's often used to predict numerical outcomes. For example, linear regression can forecast sales, demand, and prices. Regression is particularly useful in finance for predicting asset values and understanding the relationships between different financial variables.
Time Series Models
Time series models, like ARIMA, are designed to analyse data points collected or recorded at specific time intervals. These models are excellent for forecasting future values based on past trends. They account for seasonal variations and cycles, making them ideal for predicting sales during holiday seasons or stock prices over time.
Neural Networks
Neural networks are inspired by the human brain and are excellent at recognising complex patterns. They are particularly useful for handling large, multidimensional datasets. Deep learning, a subset of neural networks, is widely adopted for its ability to uncover intricate relationships between input and output variables.
Decision Trees
Decision trees use a tree-like model of decisions and their possible consequences. They are easy to understand and interpret, making them a popular choice for classification tasks. Decision trees can segment data by learning simple conditional rules, which helps in classifying new data with high accuracy.
Machine learning models are the backbone of predictive analytics, enabling businesses to make data-driven decisions and predict future outcomes with high accuracy.
Evaluating and Deploying Predictive Models
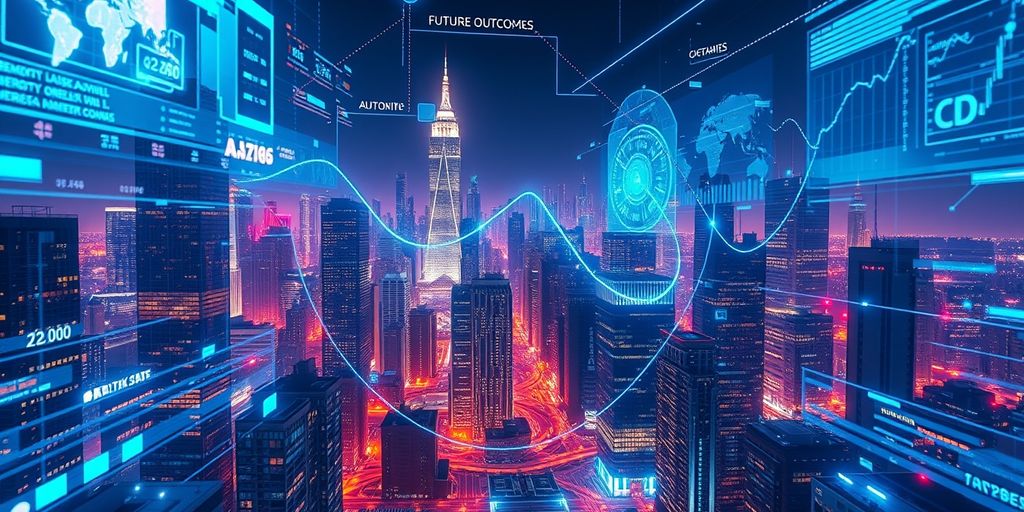
Evaluating and deploying predictive models is a crucial step in the predictive analytics process. It ensures that the models are accurate and effective in real-world applications. Let's break down the key aspects of this phase.
Model Evaluation Techniques
Before deploying a predictive model, it's essential to evaluate its performance. This involves splitting the dataset into training and test subsets to assess how well the model generalises to new data. Accuracy metrics like precision, recall, and F1 scores are used to quantify the model's performance. These metrics help in tuning the model to improve its predictive capability.
Deployment Strategies
Once a model is validated, it's time to deploy it into a production environment. This means integrating the model into business processes where it can start generating predictions from new data. For example, a predictive model in a retail setting might analyse real-time sales data to forecast future demand. Automating the input of new data and the delivery of predictions ensures that insights are readily available to decision-makers.
Continuous Monitoring and Improvement
Predictive models are not set-and-forget solutions. They require continuous monitoring and refinement based on new data and changing conditions. Regularly retraining the model with fresh data helps maintain its relevance and accuracy. Fine-tuning the model by experimenting with different configurations and parameters can significantly impact its effectiveness. Establishing a feedback mechanism to capture the model's performance in real-world applications is also crucial for ongoing improvement.
Predictive models need constant attention to stay effective. Regular updates and fine-tuning ensure they remain accurate and useful.
By following these steps, businesses can unlock the power of predictive AI for data-driven forecasting and business growth.
Future Trends in Predictive Analytics
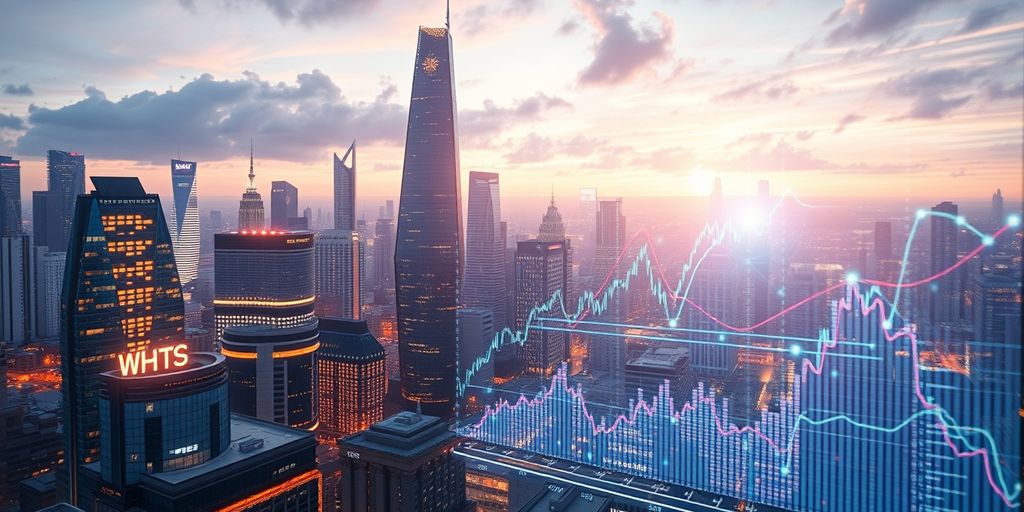
Emerging Technologies
Predictive analytics is evolving rapidly, with new technologies constantly emerging. Generative AI models are now being used to create realistic data, which can help improve predictions in areas where data is scarce. Additionally, incorporating external datasets like weather forecasts and financial indicators can enhance the accuracy of time series predictions. Quantum machine learning algorithms are also on the rise, offering faster processing speeds and allowing complex models to be updated quickly with the latest data.
Adoption Growth Trajectory
The market for predictive analytics is growing fast. Valued at over $10 billion, it is expected to grow at a compound annual growth rate (CAGR) of 22% until 2030. Industries that still rely heavily on manual decisions, such as law, education, and journalism, are likely to be transformed by data-based forecasts.
Impact on Various Industries
Predictive analytics is set to revolutionise many sectors. In healthcare, it can help with early disease detection and personalised treatment plans. In finance, algorithmic trading systems already account for over 70% of stock market trades. The potential for predictive analytics to improve efficiency and decision-making is enormous across all industries.
The future of predictive analytics is both promising and uncertain, depending on how it is developed and utilised. Responsible AI development is crucial to mitigate ethical concerns and risks.
Predictive analytics is evolving rapidly, and the future holds exciting trends that will change how we understand data. From advanced AI tools to real-time data processing, the possibilities are endless. Stay ahead of the curve by exploring these trends on our website. Don't miss out on the latest updates and insights!
Conclusion
AI-based predictive analytics is like having a crystal ball for your business. It helps you make better decisions, work more efficiently, and even spot problems before they happen. But, it's not all sunshine and rainbows. There are challenges, like making sure your data is good and keeping things fair and unbiased.
Despite these hurdles, the benefits are huge. From predicting market trends to personalising customer experiences, AI predictive analytics is changing the game. As we move forward, it's clear that this technology will play a big role in shaping the future. So, whether you're in healthcare, retail, or any other industry, embracing AI predictive analytics could be your ticket to staying ahead of the curve.
Frequently Asked Questions
What is AI-based predictive analytics?
AI-based predictive analytics uses artificial intelligence to analyse current and past data to forecast future events. It helps in making better decisions by identifying patterns and trends in the data.
How does AI improve decision-making?
AI improves decision-making by quickly processing large amounts of data to provide insights. This reduces guesswork and helps in planning more effectively.
What are the benefits of using AI-based predictive analytics?
The benefits include better decision-making, increased efficiency, proactive risk management, and the ability to personalise services and products for customers.
What challenges might one face when using AI-based predictive analytics?
Challenges include data quality issues, the complexity of models, and ethical considerations like data privacy and fairness.
How is predictive analytics used in healthcare?
In healthcare, predictive analytics is used for early disease detection, creating personalised treatment plans, predicting epidemic outbreaks, and improving diagnostic imaging.
What types of machine learning models are used in predictive analytics?
Common models include regression analysis, time series models, neural networks, and decision trees. These models help in making accurate predictions based on data.