Machine learning, a subset of artificial intelligence (AI), has transformed from a theoretical concept to a practical tool that impacts our daily lives.
From healthcare to finance, and entertainment to education, machine learning algorithms are embedded in various sectors. This article explores the historical milestones, fundamental concepts, technological breakthroughs, applications, challenges, and future prospects of machine learning.
Key Takeaways
- Machine learning has evolved significantly since its inception in the 1940s, with notable contributions from pioneers like Alan Turing and Frank Rosenblatt.
- Supervised, unsupervised, and reinforcement learning are the three main types of machine learning, each with its unique approach and application.
- Technological advancements such as deep learning and transformers have revolutionised fields like natural language processing and image recognition.
- Machine learning is widely applied across various industries, including healthcare, finance, and entertainment, driving innovation and efficiency.
- Ethical considerations, including bias, fairness, and data privacy, are crucial as machine learning continues to evolve and integrate with emerging technologies like quantum computing.
Historical Milestones in Machine Learning
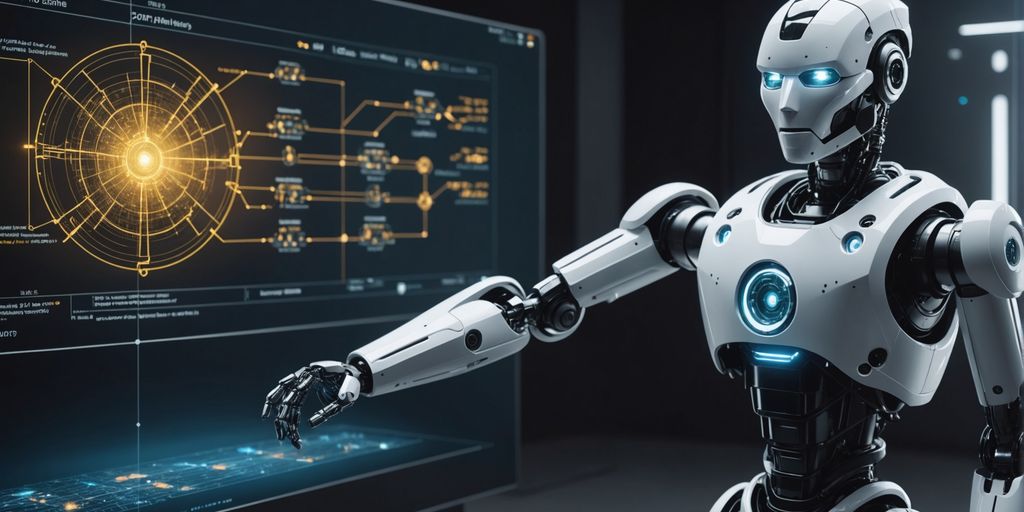
The Birth of Neural Networks
The journey of machine learning began in the 1940s. In 1943, logician Walter Pitts and neuroscientist Warren McCulloch created the first mathematical model of a neural network. This model aimed to mimic human thought processes. By 1949, Donald Hebb published a seminal book, The Organisation of Behaviour: A Neuropsychological Theory, which focused on how brain activity relates to neural networks. This work laid the groundwork for future developments in machine learning.
The Advent of the Turing Test
In 1950, Alan Turing published Computing Machinery and Intelligence, introducing the Turing Test. This test was designed to determine if a machine could exhibit intelligent behaviour equivalent to, or indistinguishable from, that of a human. The Turing Test opened the door to what would later be known as artificial intelligence (AI).
Early Pioneers and Innovations
The 1950s and 1960s saw significant contributions from pioneers like Turing, Samuel, McCarthy, Minsky, Edmonds, and Newell. During this period, the first artificial neural network and the terms artificial intelligence and machine learning were conceived. The 1960s also witnessed the development of Eliza, the first chatbot, and Shakey, the first mobile intelligent robot. These early innovations set the stage for the rapid advancements that followed in the subsequent decades.
The evolution of machine learning has been marked by groundbreaking milestones, from the creation of the first neural networks to the introduction of the Turing Test. These early achievements have paved the way for the sophisticated AI systems we see today.
Fundamental Concepts of Machine Learning
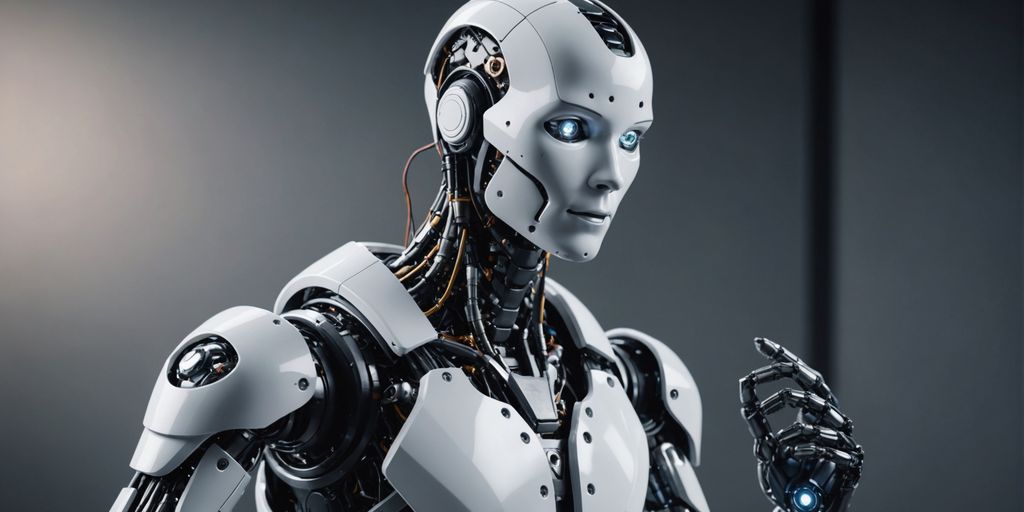
Supervised Learning Explained
Supervised learning is the most common type of machine learning. In this approach, models are trained using labelled datasets. For example, if you want a machine to recognise pictures of dogs, you would train it with many labelled images of dogs and other objects. The machine learns to identify dogs by finding patterns in these labelled images.
Unsupervised Learning Techniques
Unsupervised learning, on the other hand, deals with unlabelled data. The goal is to find hidden patterns or structures in the data without human intervention. For instance, businesses can use unsupervised learning for customer segmentation or data analysis. This type of learning is useful for discovering trends that people might not be explicitly looking for.
Reinforcement Learning Dynamics
Reinforcement learning is a bit different. It relies on a system of rewards and penalties to teach the machine. The machine learns by trial and error, aiming to maximise its rewards. This method is often used in training models to play games or drive autonomous vehicles. The machine gets feedback on its actions and learns the best strategies over time.
Machine learning is a subset of AI where the machine is trained to learn from its past experience. The past experience is developed through the data collected.
Breakthroughs in Machine Learning Technology
The Rise of Deep Learning
Deep learning has revolutionised the field of machine learning by enabling computers to recognise patterns in data such as images, text, and audio. A pivotal moment came in 2012 when AlexNet, a convolutional neural network, outperformed traditional methods in the ImageNet competition. This victory marked a decisive shift towards deep learning architectures, which have since been applied in various industries, from healthcare to entertainment.
Generative Adversarial Networks
In 2014, Ian Goodfellow introduced Generative Adversarial Networks (GANs), a groundbreaking technology that allows for the generation of realistic synthetic data. GANs consist of two neural networks, a generator and a discriminator, that compete against each other to improve the quality of the generated data. This innovation has opened new avenues in fields such as art, fashion, and even drug discovery.
Transformers and Natural Language Processing
The introduction of transformer models in 2017 revolutionised natural language processing (NLP). Transformers enable machines to understand and generate human language with unprecedented accuracy. These models have been the backbone of many advanced NLP applications, including chatbots, translation services, and sentiment analysis. The impact of transformers extends beyond NLP, influencing other areas like computer vision and reinforcement learning.
The advancements in machine learning technology have not only transformed industries but also paved the way for future innovations. As we continue to explore the potential of these technologies, the importance of understanding their implications cannot be overstated.
Applications of Machine Learning in Various Industries
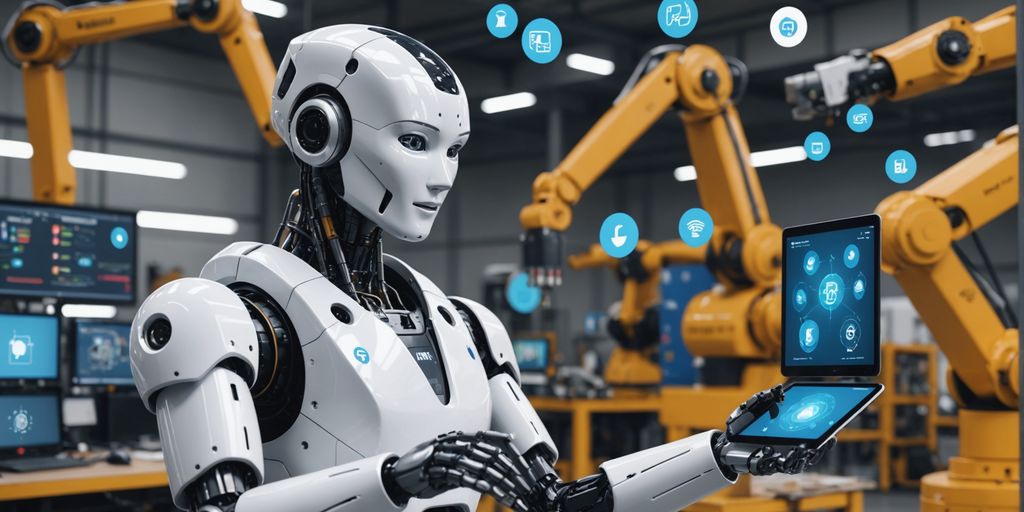
Healthcare Innovations
Machine learning is transforming healthcare by enabling predictive analytics and personalised treatment plans. It helps in analysing medical images, such as X-rays and MRIs, to detect diseases early. Additionally, machine learning algorithms can predict patient outcomes, improving the overall quality of care.
Financial Sector Advancements
In the financial sector, machine learning is used for fraud detection, risk management, and financial forecasting. By analysing patterns in data, these algorithms can identify unusual activities, helping to prevent fraud. Moreover, machine learning models can predict market trends, aiding in better investment decisions.
Impact on Entertainment and Media
Machine learning powers recommendation systems on platforms like Netflix and Spotify, providing personalised content to users. It also plays a role in content creation and curation, making it easier for media companies to deliver relevant content to their audiences. Furthermore, machine learning is used in analysing user behaviour to improve engagement and retention.
Machine learning is revolutionising various industries by automating complex processes and making intelligent predictions or decisions by “digesting” vast amounts of information.
Challenges and Ethical Considerations
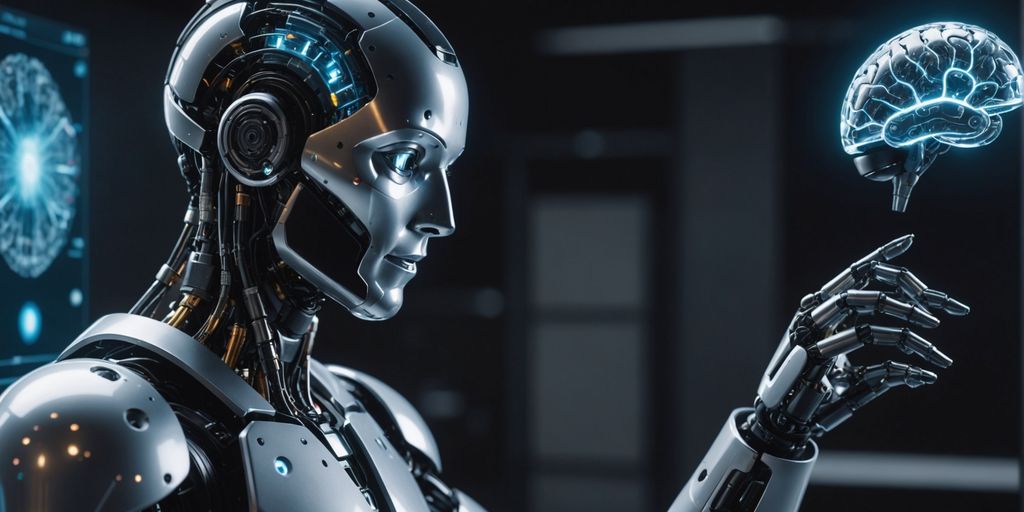
Bias and Fairness in Algorithms
Machine learning systems can sometimes show biases that reflect the data they were trained on. For instance, if a hiring algorithm is trained on data from a company with biassed hiring practises, it might continue those biases. This can lead to unfair treatment of certain groups. To fight against this, it's important to carefully check the training data and make sure it is fair.
Data Privacy Concerns
Data privacy is a big issue in machine learning. Systems often need a lot of data to learn, but using personal data can lead to privacy problems. It's important to make sure that data is used responsibly and that people's privacy is protected. This includes following laws and guidelines about data use.
The Future of Responsible AI
As machine learning continues to grow, it's important to think about how it will be used in the future. This includes making sure that AI is used in a way that is fair and ethical. Organisations need to support efforts to create responsible AI, like making sure their systems are designed with input from people of different backgrounds. This can help prevent problems and make sure that AI is used for good.
The Future of Machine Learning
Integration with Quantum Computing
Quantum computing is set to revolutionise the field of machine learning. By leveraging the principles of quantum mechanics, quantum computers can process vast amounts of data at unprecedented speeds. This will enable machine learning models to solve complex problems that are currently beyond the reach of classical computers. The integration of quantum computing with machine learning could lead to breakthroughs in areas such as cryptography, optimisation, and drug discovery.
Advancements in Self-Learning Systems
Self-learning systems are designed to improve their performance over time without human intervention. These systems use techniques such as reinforcement learning and unsupervised learning to adapt to new data and environments. As these technologies advance, we can expect to see more autonomous systems capable of making decisions and learning from their experiences. This will have significant implications for industries such as autonomous vehicles, robotics, and personalised medicine.
Potential Societal Impacts
The future of machine learning holds great promise, but it also raises important ethical and societal questions. Issues such as bias in algorithms, data privacy, and the impact on employment need to be carefully considered. It is crucial to develop responsible AI practises to ensure that the benefits of machine learning are shared equitably. As we move forward, it will be essential to engage in ongoing dialogue about the ethical implications of these technologies and to develop frameworks for their responsible use.
The future of machine learning is not just about technological advancements; it is also about ensuring that these advancements are used for the greater good. We must strive to create a future where machine learning benefits everyone and contributes to a more just and equitable society.
Machine learning is changing fast, and its future looks bright. From self-driving cars to smart assistants, the possibilities are endless. Want to stay updated on the latest in AI and machine learning? Visit our website for the newest trends and insights. Don't miss out on the future!
Conclusion
Machine learning has come a long way since its early days in the 1940s. From simple algorithms that could play games to advanced systems that can drive cars and diagnose diseases, the journey has been remarkable. Today, machine learning is a crucial part of many industries, helping to solve complex problems and improve our daily lives.
As we look to the future, the potential for machine learning is immense. With continued research and development, we can expect even more innovative applications that will shape the world in ways we can only imagine. The story of machine learning is far from over; it's just beginning.
Frequently Asked Questions
What is machine learning?
Machine learning is a type of artificial intelligence where computers learn from data and improve their performance over time without being explicitly programmed.
How does supervised learning work?
In supervised learning, the computer is trained using a set of data that includes both the input and the desired output. The system learns to map the input to the output and can then make predictions on new data.
What is the difference between supervised and unsupervised learning?
In supervised learning, the model is trained on labelled data, meaning the input comes with the correct output. In unsupervised learning, the model is given data without labelled responses and must find patterns and relationships within the data.
What are some applications of machine learning in healthcare?
Machine learning is used in healthcare for tasks like diagnosing diseases from medical images, predicting patient outcomes, personalising treatment plans, and analysing patient data for research.
Why is data privacy a concern in machine learning?
Data privacy is a concern because machine learning models often require large amounts of personal data to function effectively. This data needs to be protected to prevent misuse and ensure individuals' privacy is maintained.
What is the future of machine learning?
The future of machine learning includes advancements like integration with quantum computing, more sophisticated self-learning systems, and broader societal impacts, including both positive innovations and ethical challenges.