Artificial Intelligence (AI) has come a long way from its early beginnings.
Today, it plays a pivotal role in various aspects of our lives, from healthcare to education. This article explores the journey of AI, its integration with cognitive reasoning, and the implications of these advancements on society. We will also discuss the importance of understanding how AI can work alongside human intelligence to enhance decision-making and problem-solving capabilities.
Key Takeaways
AI has evolved significantly, thanks to key milestones and contributions from researchers.
Cognitive reasoning allows AI systems to make informed decisions, similar to human thinking.
Machine learning is a crucial part of AI, enabling systems to learn from data and improve over time.
Human-machine collaboration is essential for optimising the strengths of both AI and human intelligence.
Ethical considerations are vital in AI development to ensure fairness and transparency.
The Evolution of Artificial Intelligence
Historical Milestones in AI Development
Artificial intelligence has come a long way since its inception. Here are some key milestones:
1956: The term "artificial intelligence" was coined at the Dartmouth Conference.
1997: IBM's Deep Blue defeated world chess champion Garry Kasparov.
2011: IBM's Watson won the quiz show Jeopardy!
Key Contributors to AI Research
Several individuals have played crucial roles in the development of AI:
John McCarthy: Often referred to as the father of AI.
Alan Turing: Developed the Turing Test to measure a machine's ability to exhibit intelligent behaviour.
Marvin Minsky: Co-founder of the MIT AI Lab and a pioneer in AI research.
Technological Advancements Driving AI
The growth of AI has been supported by various technological advancements:
Increased computing power: More powerful processors allow for complex calculations.
Big data: The availability of vast amounts of data enables better training of AI models.
Machine learning algorithms: These algorithms help AI systems learn from data and improve over time.
The journey of artificial intelligence is marked by significant achievements and ongoing challenges. As we continue to explore its potential, the integration of AI into various sectors is becoming increasingly vital.
In summary, the evolution of artificial intelligence is a fascinating story of innovation, driven by key milestones, influential figures, and technological advancements. AI is reshaping our world, and understanding its history helps us appreciate its future possibilities.
Cognitive Reasoning in AI Systems
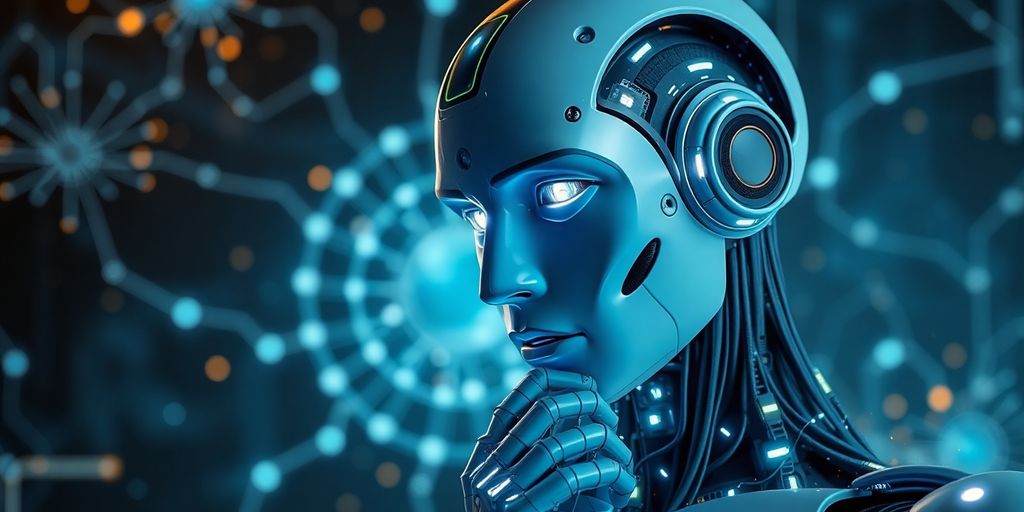
Understanding Cognitive Reasoning
Cognitive reasoning refers to the processes that allow AI systems to think and make decisions like humans. This involves understanding information, drawing conclusions, and solving problems. AI systems use various techniques to mimic human thought processes, enabling them to tackle complex tasks effectively.
Integration of Cognitive Reasoning and AI
The integration of cognitive reasoning into AI systems has led to significant advancements. By combining these two fields, we can create systems that:
Understand context better, improving their responses.
Make informed decisions based on available data.
Learn from experiences, enhancing their reasoning capabilities over time.
Challenges in Implementing Cognitive Reasoning
Despite the progress, there are still challenges in implementing cognitive reasoning in AI:
Data Quality: AI systems require high-quality data to reason effectively.
Complexity of Human Thought: Mimicking human reasoning is difficult due to its complexity.
Ethical Concerns: Ensuring that AI systems make fair and unbiased decisions is crucial.
The intersection of artificial intelligence and cognitive reasoning offers profound insights into the nature of human knowledge and thought.
In summary, cognitive reasoning is a vital aspect of AI systems, enabling them to perform tasks that require human-like thinking. As technology advances, the integration of these concepts will continue to evolve, presenting both opportunities and challenges for the future of AI.
Machine Learning and Its Role in AI
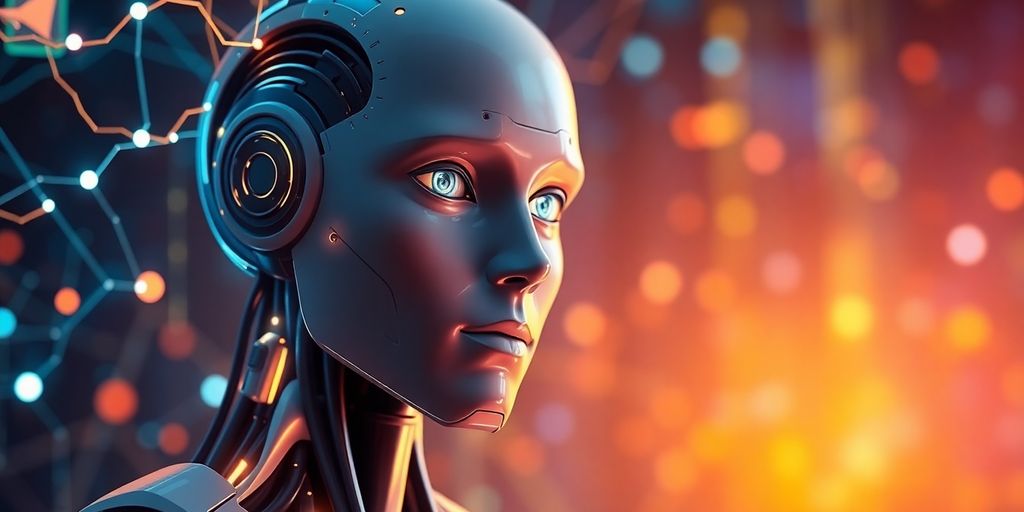
Fundamentals of Machine Learning
Machine Learning (ML) is a crucial part of artificial intelligence. It focuses on teaching computers to learn from data and improve over time without being explicitly programmed. The most commonly discussed sub-set is machine learning (ML), which is specifically about applying complex algorithms and statistical techniques to existing data. Here are some key concepts:
Supervised Learning: The model learns from labelled data.
Unsupervised Learning: The model identifies patterns in unlabelled data.
Reinforcement Learning: The model learns by receiving rewards or penalties.
Applications of Machine Learning in AI
Machine learning has numerous applications that enhance AI capabilities. Some notable examples include:
Natural Language Processing: Understanding and generating human language.
Computer Vision: Enabling machines to interpret and understand visual information.
Predictive Analytics: Making predictions based on historical data.
Application | Description |
---|---|
Healthcare | Assisting in diagnostics and treatment plans. |
Finance | Fraud detection and risk assessment. |
Autonomous Vehicles | Navigating and making decisions on the road. |
Future Trends in Machine Learning
The future of machine learning looks promising, with several trends emerging:
Explainable AI: Making AI decisions understandable to humans.
Federated Learning: Training models across multiple devices without sharing data.
Ethical AI: Ensuring fairness and transparency in AI systems.
The integration of machine learning with cognitive reasoning is transforming how AI systems operate, making them more efficient and capable of complex decision-making.
In summary, machine learning plays a vital role in the development of AI, enabling systems to learn, adapt, and improve their performance in various applications.
Human-Machine Collaboration
Balancing Human and Artificial Cognition
In today's world, the partnership between humans and machines is becoming increasingly important. Each has unique strengths:
Humans excel in creativity, emotional understanding, and complex decision-making.
Machines are great at processing large amounts of data quickly and accurately.
This balance allows for more effective problem-solving and innovation.
Enhancing Human-Machine Interfaces
To improve collaboration, we need better interfaces that allow humans and machines to work together seamlessly. Some key features include:
User-friendly designs that are easy to navigate.
Natural language processing to understand human commands.
Adaptive systems that learn from user behaviour to improve interactions.
These advancements can lead to more productive and satisfying experiences for users.
Ethical Considerations in Human-Machine Collaboration
As we integrate AI into our daily lives, we must consider the ethical implications:
Fairness: Ensuring AI systems do not discriminate.
Transparency: Making AI decisions understandable to users.
Accountability: Establishing who is responsible for AI actions.
The future of human-machine collaboration relies on trust and understanding between both parties. By addressing these ethical concerns, we can create a more harmonious relationship.
In summary, the collaboration between humans and machines is essential for progress. By leveraging the strengths of both, we can tackle complex challenges and enhance our capabilities.
AI in Real-World Applications
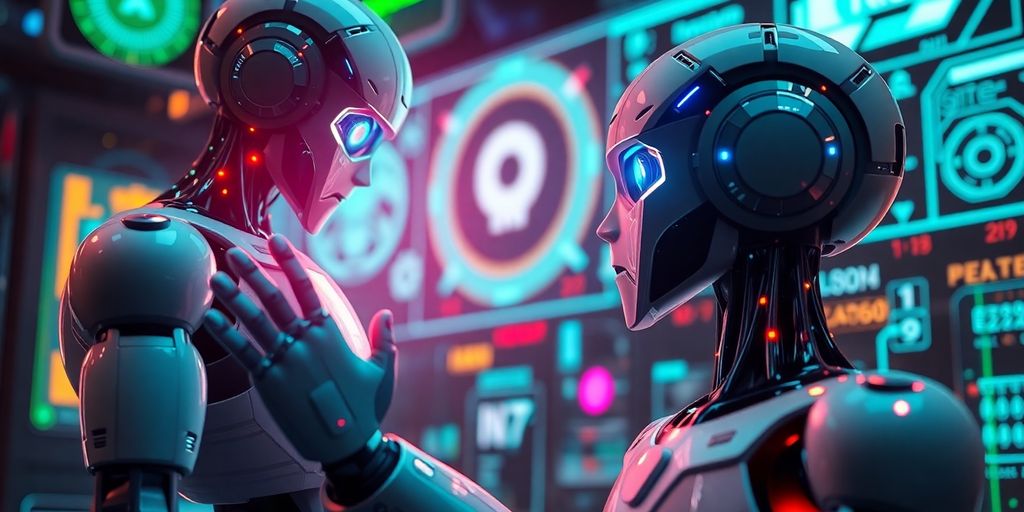
Artificial Intelligence (AI) is making a significant impact across various sectors, transforming how we live and work. AI technologies are now integral to many everyday applications, enhancing efficiency and decision-making.
AI in Healthcare and Diagnostics
AI is revolutionising healthcare by:
Improving diagnostics through image recognition and analysis.
Predicting patient outcomes using data analytics.
Personalising treatment plans based on individual patient data.
AI in Natural Language Processing
Natural Language Processing (NLP) allows machines to understand and respond to human language. Key applications include:
Chatbots for customer service.
Language translation tools that break down communication barriers.
Sentiment analysis to gauge public opinion on social media.
AI in Autonomous Systems
Autonomous systems, such as self-driving cars, are becoming more prevalent. They rely on AI to:
Navigate complex environments using real-time data.
Make decisions based on predictive analytics.
Enhance safety by reducing human error.
AI is not just a futuristic concept; it is already shaping our daily lives and industries. Its ability to analyse vast amounts of data enables better decision-making and efficiency.
In summary, AI is a powerful tool that is reshaping various fields, from healthcare to transportation, and its potential continues to grow. As we explore these applications, it is essential to consider the ethical implications and ensure responsible use of AI technologies.
Sector | Key Benefits |
---|---|
Healthcare | Improved diagnostics, personalised care |
Natural Language Processing | Enhanced communication, sentiment analysis |
Autonomous Systems | Increased safety, reduced human error |
AI's role in these areas highlights its transformative power, making it a crucial component of modern technology.
Ethical and Societal Implications of AI
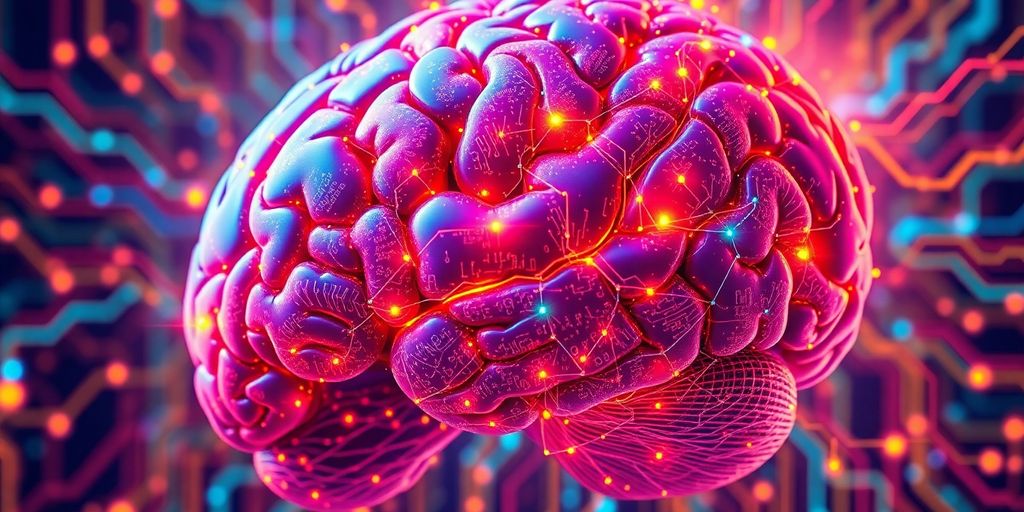
Ensuring Fairness and Transparency
The rapid growth of AI technology raises important questions about fairness and transparency. As AI systems are increasingly used in decision-making processes, it is crucial to ensure that these systems do not perpetuate existing biases. Here are some key points to consider:
Bias in Data: AI systems learn from data, and if the data contains biases, the AI will likely reflect those biases in its decisions.
Transparency: Users should understand how AI systems make decisions. This can help build trust and accountability.
Collaboration: Policymakers, businesses, and individuals must work together to create guidelines that promote responsible AI use.
Addressing Privacy Concerns
With the rise of AI, privacy concerns have become more prominent. AI systems often require large amounts of data, which can lead to potential misuse. Here are some considerations:
Data Protection: Implementing strong data protection measures is essential to safeguard personal information.
User Consent: Users should have control over their data and be informed about how it is used.
Regulatory Frameworks: Establishing clear regulations can help protect individuals from data exploitation.
The Future of Work with AI
AI is transforming the workplace, leading to both opportunities and challenges. The impact of AI on jobs is a significant concern:
Job Displacement: Some jobs may be replaced by AI, leading to unemployment in certain sectors.
New Opportunities: Conversely, AI can create new job roles that require different skills.
Skill Development: Continuous learning and adaptation will be necessary for workers to thrive in an AI-driven economy.
The rise of artificial intelligence in modern technology presents both exciting opportunities and significant challenges. It is essential to navigate these changes thoughtfully to ensure a positive impact on society.
Advancements in AI Algorithms
Deep Learning Techniques
Deep learning has revolutionised the field of AI by enabling systems to learn from vast amounts of data. This approach mimics the way humans learn, using neural networks to process information. Key aspects include:
Layered architecture: Deep learning models consist of multiple layers that help in feature extraction.
Data-driven: These models require large datasets to improve accuracy.
Applications: Used in image recognition, speech processing, and more.
Reinforcement Learning Innovations
Reinforcement learning (RL) is a type of machine learning where agents learn to make decisions by receiving rewards or penalties. This method has shown great promise in various fields. Some highlights are:
Game playing: RL has been used to develop AI that can play complex games like chess and Go.
Robotics: Robots can learn to perform tasks through trial and error.
Real-world applications: RL is being applied in areas like finance and healthcare for optimising decisions.
Probabilistic Models and Bayesian Approaches
Probabilistic models help AI systems make predictions based on uncertainty. Bayesian approaches allow for updating beliefs as new data comes in. Important points include:
Flexibility: These models can adapt to new information.
Decision-making: They assist in making informed choices under uncertainty.
Applications: Used in fields like weather forecasting and medical diagnosis.
The integration of these advancements is crucial for developing smarter AI systems that can reason and adapt to complex environments. Understanding these algorithms is essential for harnessing AI's full potential.
Artificial Intelligence (AI) is making great strides, changing how we think and work. With new algorithms, AI is becoming smarter and more efficient, helping us solve complex problems faster than ever. If you're curious about the latest developments in AI, visit our website for more insights and updates!
Conclusion
In summary, the relationship between artificial intelligence and cognitive reasoning is crucial for the future of technology. As AI continues to develop, it is becoming more adept at mimicking human thought processes, which enhances its ability to solve problems and make decisions. This partnership not only improves how machines interact with us but also opens up new possibilities for various fields, including education and healthcare.
However, it is essential to remember that while AI can perform many tasks efficiently, it lacks the emotional intelligence and creativity that humans possess. Therefore, the best outcomes arise when humans and machines work together, each contributing their unique strengths. As we move forward, understanding and improving this collaboration will be key to unlocking the full potential of artificial intelligence.
Frequently Asked Questions
What is Artificial Intelligence (AI)?
Artificial Intelligence (AI) is when computers are designed to think and act like humans. It helps machines make decisions and complete tasks.
How does Machine Learning relate to AI?
Machine Learning is a part of AI that teaches computers to learn from data. It helps improve AI's ability to make decisions over time.
What is Cognitive Reasoning in AI?
Cognitive Reasoning in AI refers to how machines can understand, think, and make decisions, similar to how humans do.
What are the benefits of AI in everyday life?
AI can make daily tasks easier, like helping with scheduling, providing recommendations, and even assisting in healthcare.
What challenges do we face with AI?
Some challenges include ensuring AI is fair, protecting privacy, and making sure it doesn't replace human jobs.
How can AI and humans work together?
AI can assist humans by taking over repetitive tasks, allowing people to focus on more complex and creative work.