A groundbreaking AI algorithm developed by researchers at the University of Southern California (USC) has the potential to revolutionise our understanding of brain activity related to specific behaviours. This innovative technology, known as DPAD (Dissociative Prioritised Analysis of Dynamics), can effectively separate and identify brain patterns associated with particular actions, paving the way for advancements in brain-computer interfaces and mental health treatments.
Key Takeaways
New AI algorithm can identify specific brain patterns related to behaviours.
Developed by Maryam Shanechi and her team at USC.
Enhances brain-computer interfaces for paralysed patients.
Potential applications in mental health treatment.
Understanding Brain Activity
As individuals engage in various activities, their brains simultaneously process multiple behaviours. For instance, while reaching for a cup of coffee, one might also be reading or feeling hungry. This complexity creates intricate patterns in the brain's electrical activity, making it challenging to isolate the specific patterns that correspond to a single behaviour.
The ability to dissociate these patterns is crucial for developing effective brain-computer interfaces (BCIs). These devices aim to restore movement in paralysed patients by decoding their intended movements directly from brain activity and translating them into actions, such as controlling a robotic arm or a computer cursor.
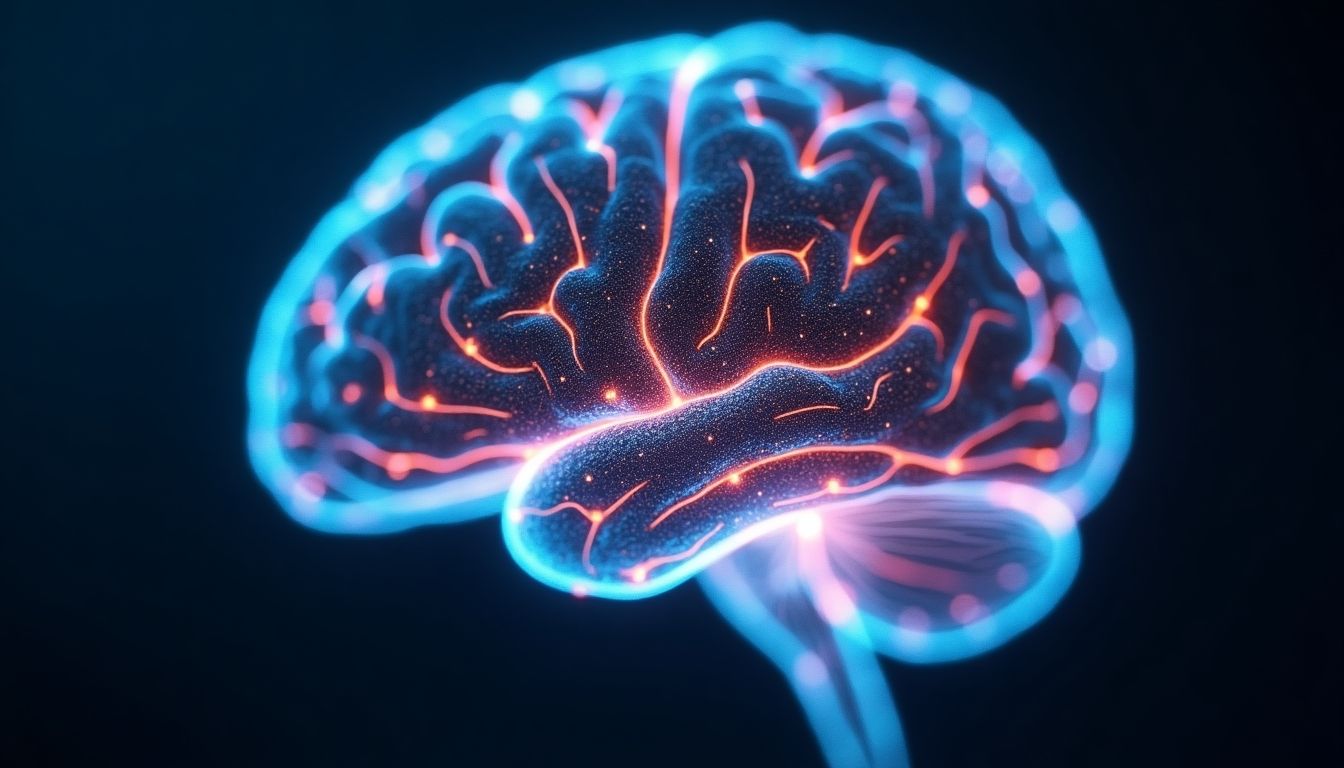
The DPAD Algorithm
The DPAD algorithm, created by Maryam Shanechi and her former Ph.D. student Omid Sani, addresses the challenge of identifying behaviour-specific brain patterns. The key features of DPAD include:
Prioritised Learning: The algorithm focuses on identifying brain patterns related to the behaviour of interest during the training of a deep neural network.
Flexibility: It can learn and describe various types of brain patterns, allowing for a broader application in different contexts.
Enhanced Accuracy: By effectively dissociating behaviour-related patterns from other simultaneous brain activities, DPAD improves the accuracy of movement decoding compared to previous methods.
Future Implications
Beyond movement, the DPAD algorithm holds promise for decoding mental states, such as pain or depression. This capability could significantly enhance the treatment of mental health conditions by providing real-time feedback on a patient's symptoms, allowing for more tailored therapeutic approaches.
Maryam Shanechi expressed her enthusiasm for the potential extensions of their method, stating, "We are very excited to develop and demonstrate extensions of our method that can track symptom states in mental health conditions. Doing so could lead to brain-computer interfaces not only for movement disorders and paralysis but also for mental health conditions."
Conclusion
The development of the DPAD algorithm marks a significant milestone in neuroscience and artificial intelligence. By enabling the identification of specific brain patterns related to behaviour, this innovative technology could transform the landscape of brain-computer interfaces and mental health treatment, offering new hope for individuals with movement disorders and mental health challenges alike.