Artificial intelligence is everywhere these days, from chatbots to recommendation systems.
But there's a big issue lurking in the background—bias and fairness. These aren't just buzzwords; they really matter. When AI systems are biassed, they can make unfair decisions, leading to real-world harm. So, how do we tackle this? It's all about understanding where bias comes from and how we can make AI systems fairer. Let's dive into the nitty-gritty of bias and fairness in AI, and explore ways to make AI work for everyone.
Key Takeaways
Bias in AI can lead to unfair outcomes, affecting real-world decisions.
Ensuring fairness in AI requires conscious efforts, like diverse data collection and regular monitoring.
Governance and ethical guidelines play a crucial role in maintaining AI fairness.
Understanding Bias in Artificial Intelligence
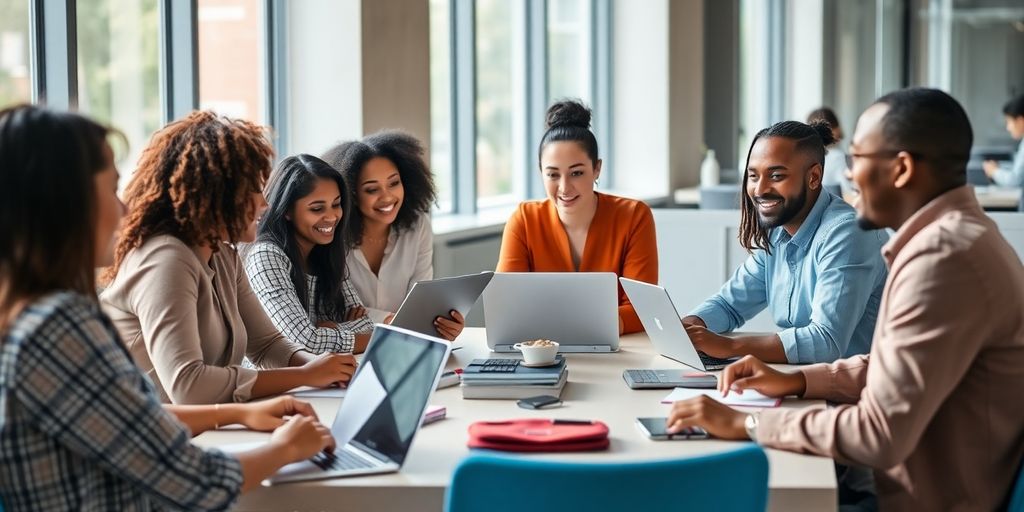
Defining Bias in AI Systems
Bias in AI, often called algorithmic bias, refers to systematic errors that skew results unfairly. These biases can creep in from various stages of an AI system's lifecycle, like data collection, algorithm design, or even human biases. AI algorithms can learn and amplify human biases present in the data, which can enhance their prediction accuracy. This is a big deal because it can lead to skewed outcomes, affecting everything from who gets a loan to what ads you see online.
Sources of Bias in AI
Bias in AI can come from a few different places:
Data Bias: If the data used to train AI isn't representative of the real world, the AI's decisions can end up being biassed.
Human Bias: The people who design and train AI systems can unintentionally introduce their own biases.
Algorithmic Bias: The way algorithms are designed can sometimes favour certain outcomes over others.
Impact of Bias on Decision-Making
When biases seep into AI systems, they can lead to decisions that aren't fair or just. For instance, biased AI in hiring can favour certain groups over others, reinforcing societal inequalities. This not only affects individuals but can also lead to broader social issues, like reduced diversity in workplaces or unfair treatment in the justice system.
In the world of AI, the presence of bias can transform what should be objective decision-making into a perpetuation of existing inequalities.
Examples of Bias in AI Applications
Bias in AI isn't just a theoretical problem—it's happening right now:
Facial Recognition: Some systems are less accurate at identifying people of certain races.
Hiring Algorithms: AI tools used in recruitment might favour candidates from specific backgrounds.
Predictive Policing: These systems can disproportionately target certain communities, leading to unfair treatment.
Understanding and addressing these biases is crucial for creating fair and equitable AI systems.
Ensuring Fairness in AI Systems
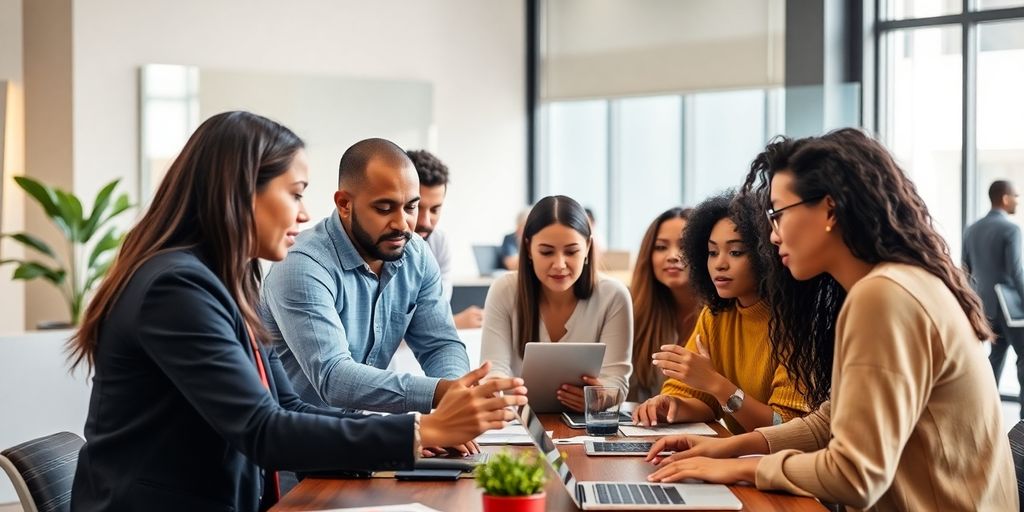
Principles of Fairness in AI
AI fairness is about making sure systems operate without bias and discrimination. It's a bit like refereeing a game; you want to be fair to all players, regardless of their background. In AI, this means designing systems that don’t favour or disadvantage anyone based on characteristics like race, gender, or socioeconomic status. Fairness is crucial for trust and acceptance of AI technologies, especially as we integrate them into everyday life.
Types of Fairness in AI
There are several approaches to fairness in AI:
Group Fairness: Ensures that different groups are treated equally. Think of it like making sure all teams in a league have the same chance of winning.
Individual Fairness: Similar individuals should be treated similarly, regardless of their group. It's like saying two players with the same skills should be given equal opportunities.
Counterfactual Fairness: AI decisions should remain fair even in hypothetical scenarios. Imagine if you could change one thing about a player, and it shouldn’t affect the fairness of their treatment.
Challenges in Achieving Fairness
Achieving fairness in AI isn't straightforward. Here are a few hurdles:
Data Bias: If the data used to train AI is biassed, the outcomes will likely be biassed too.
Complexity of Fairness: Fairness can be subjective and varies across cultures and contexts.
Resource Limitations: Ensuring fairness requires significant resources, from diverse datasets to expert oversight.
Strategies for Promoting Fairness
To promote fairness, we can adopt several strategies:
Diverse Data Collection: Use a wide range of data from various demographics to minimise bias.
Regular Audits: Continuously monitor AI systems to identify and rectify biases.
Transparent Processes: Maintain clear and open processes for AI decision-making, so stakeholders understand how decisions are made.
Fairness in AI is not just about doing the right thing; it impacts trust, legal compliance, and ultimately, the success of AI systems. As AI continues to evolve, addressing these issues becomes even more pressing.
Techniques for Mitigating Bias and Enhancing Fairness
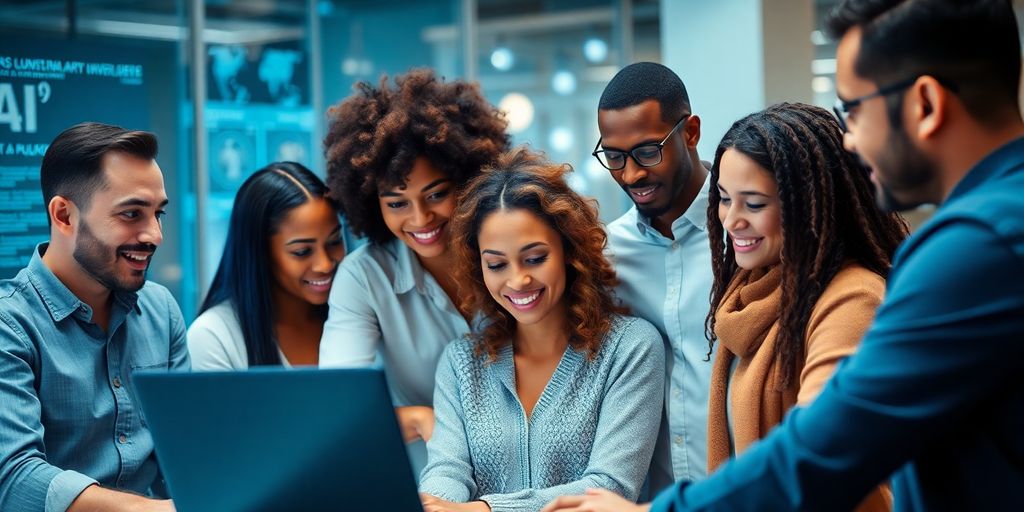
Data Collection and Preprocessing
Collecting diverse and representative data is the first step in addressing bias. Ensuring a wide range of demographics in your dataset can help reduce skewed outcomes. Preprocessing techniques involve cleaning and balancing the data, removing any potential sources of bias before they influence the model. This might include augmenting underrepresented groups in the dataset or correcting historical biases present in the data.
Algorithmic Adjustments
Algorithmic fairness isn't just a buzzword; it's about tweaking models to ensure fairer outcomes. Techniques such as re-weighting, re-sampling, or adjusting the cost functions can help mitigate bias. In-processing and post-processing methods can also be applied, like modifying the learning algorithm or adjusting the model’s outputs to align with fairness metrics.
Monitoring and Evaluation
Regular monitoring of AI systems is crucial. Implementing fairness metrics allows for ongoing evaluation of how the AI performs across different groups. This includes checking for disparate impacts or unintentional biases that may have crept in over time. Evaluation should be a continuous process, ensuring that the AI remains fair and unbiased as it evolves.
Feedback and Continuous Improvement
AI systems should be designed to learn from their mistakes. Feedback loops can be established where the AI system's outputs are reviewed, and adjustments are made based on real-world outcomes. This ongoing refinement ensures that the AI adapts and improves its fairness and bias mitigation strategies over time.
Building fair AI is not a one-time task but a continuous journey. It requires vigilance, adaptability, and a commitment to ethical standards. By focusing on these techniques, we can move towards AI systems that are both fair and unbiased, benefiting society as a whole.
The Role of Governance and Regulation in AI Fairness
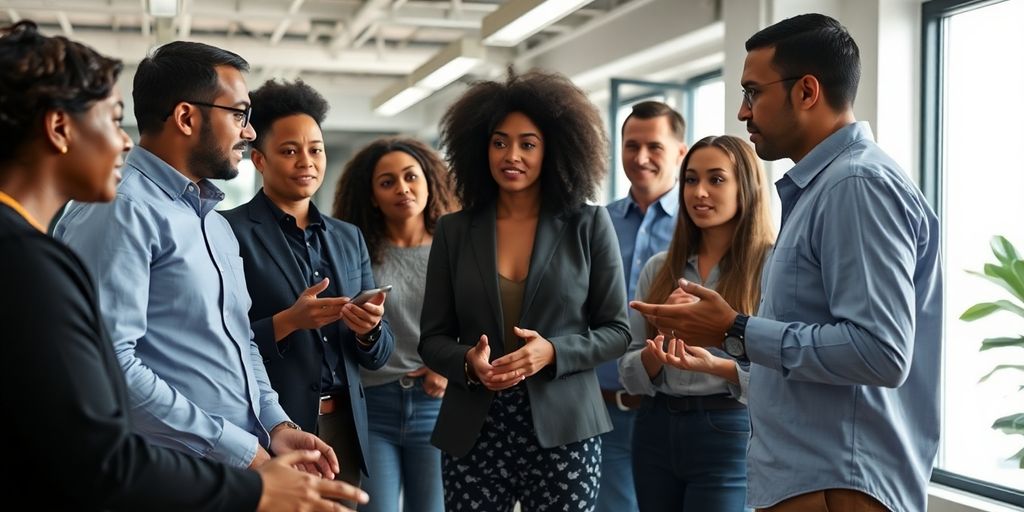
Establishing Ethical Guidelines
Creating ethical guidelines for AI is like setting the rules of the game. We need to make sure AI systems are developed and used in ways that respect human rights and societal norms. Strong governance frameworks are essential. They help ensure AI operates safely and ethically. These frameworks include oversight and accountability measures to keep AI systems fair and unbiased. AI governance is crucial for establishing these guidelines.
Legal Frameworks for AI Fairness
Legal frameworks are the backbone of ensuring fairness in AI. They provide the necessary rules and regulations that AI systems must follow. This includes compliance with data protection laws, which already emphasise fairness and accountability. AI introduces new challenges, but these frameworks are adapting to meet them. They ensure AI systems are not just technically sound but also legally compliant.
The Importance of Transparency
Transparency is key when it comes to AI. Users must understand how AI systems make decisions. This involves clear documentation and communication about AI processes. Transparency builds trust and allows for more effective monitoring of AI systems. It also helps in identifying and correcting biases, ensuring the systems remain fair over time.
Case Studies of Governance in Action
Looking at real-world examples helps us understand how governance and regulation work in practice. Case studies can show the impact of strong governance on AI fairness. They highlight successes and challenges, providing valuable lessons for future AI development. These examples demonstrate how ethical guidelines and legal frameworks are applied in real scenarios, ensuring AI systems operate fairly and responsibly.
Governance and regulation are not just about setting rules. They are about creating an environment where AI can thrive while respecting human values and rights. Through effective governance, we can ensure AI is a force for good, benefiting society as a whole.
Governance and regulation play a crucial part in ensuring fairness in artificial intelligence. By setting clear rules and guidelines, we can help make sure that AI systems treat everyone equally and do not favour one group over another. It’s important for everyone to get involved in this conversation. Visit our website to learn more about how you can contribute to a fairer AI future!
Conclusion
So, there you have it. Tackling bias and fairness in AI isn't just a techie problem; it's something we all need to think about. Sure, it's complicated, and there's no magic fix, but that doesn't mean we shouldn't try. By being aware of where bias sneaks in and making fairness a priority, we can build AI systems that are more just and trustworthy. It's about making sure AI works for everyone, not just a select few.
As we move forward, keeping these issues in mind will help us create a future where AI is a tool for good, not a source of inequality. Let's keep the conversation going and make sure we're all part of the solution.