The rapid rise of artificial intelligence, particularly with the advent of OpenAI's ChatGPT, has transformed the tech landscape. However, as leading developers like OpenAI, Google, and Anthropic face challenges in improving their models, the industry is at a crossroads, questioning the sustainability and profitability of AI advancements.
Key Takeaways
The initial excitement around AI tools like ChatGPT has led to significant investments.
Progress in AI development is becoming increasingly challenging and costly.
The concept of synthetic data is emerging as a potential solution for training AI models.
The future of AI profitability remains uncertain, with companies struggling to justify their expenses.
The Origin Of The AI Boom
AI's journey began in the 1950s, with pioneers like Alan Turing laying the groundwork. The recent surge in interest can be traced back to breakthroughs in large language models (LLMs), particularly with the launch of ChatGPT. This tool not only captured public imagination but also attracted substantial investment, as tech companies bet on the continued sophistication of AI systems.
The Limits Of Large Language Models
Despite the initial success, the development of LLMs is hitting a plateau. Companies are discovering that simply feeding more data and computational power into these models does not guarantee improved performance. As expenses rise, the industry is grappling with the reality that the low-hanging fruit of AI advancements may have been picked clean.
The Challenge Of Data Scarcity
As AI models become more complex, the demand for high-quality data increases. The internet has largely been scraped for data, making it difficult to find new, curated datasets. Companies are now resorting to hiring experts to generate the necessary data, which adds to the costs and complexity of AI development.
Exploring Synthetic Data
In response to data scarcity, some projects are experimenting with synthetic data—using AI-generated content to train new models. While this approach shows promise, it is still in the testing phase, and its reliability remains uncertain. Companies must balance the use of synthetic data with the need for high-quality, human-generated datasets.
Cost Pressures In The AI Industry
The financial implications of AI development are staggering. Training a new AI model can cost upwards of a hundred million dollars, with projections suggesting that this could rise to a hundred billion in the coming years. As companies invest heavily, the engineering challenges also multiply, necessitating larger teams and more resources.
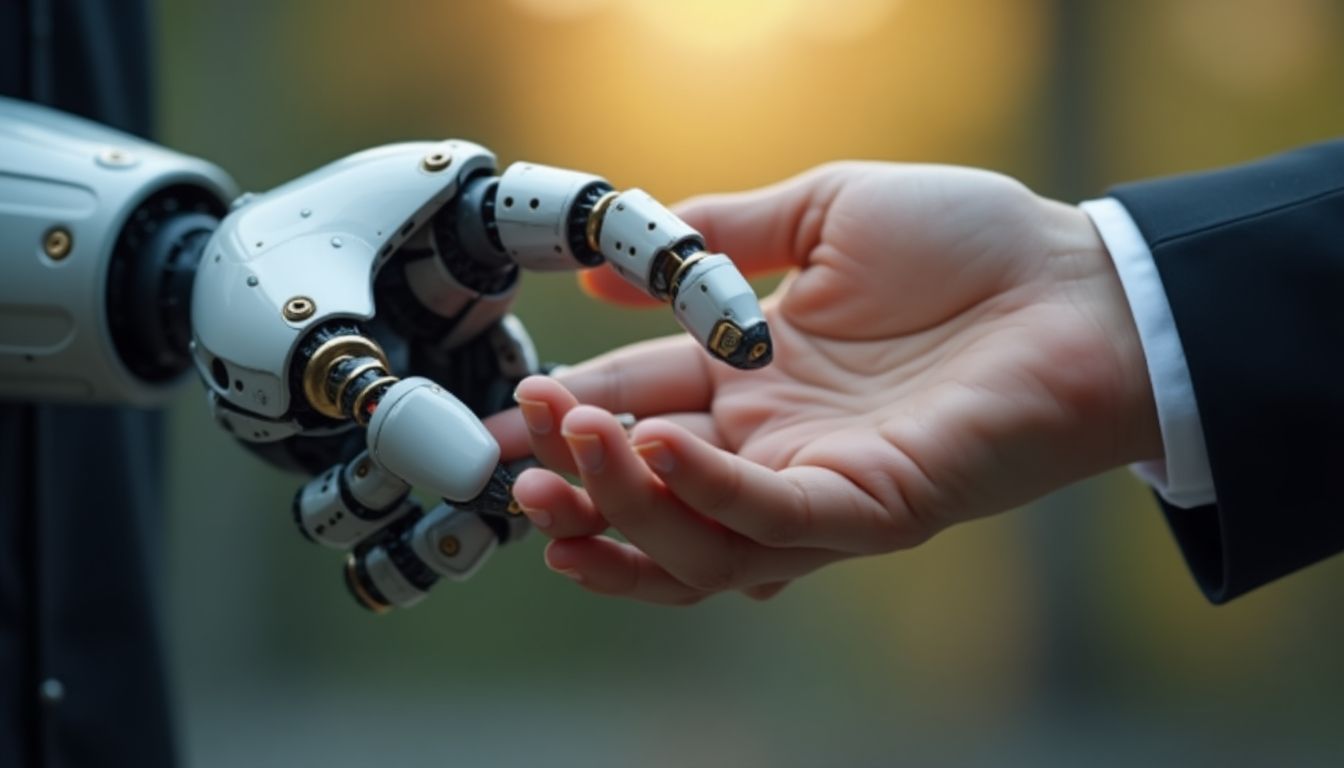
The Profitability Question
Despite the rapid growth of AI tools like ChatGPT, the question of profitability looms large. While OpenAI has secured numerous paying business customers, the exact financial benefits for these companies remain unclear. The challenge lies in balancing the costs of development with the returns on investment, a task that is proving increasingly difficult.
The Future Of AI
Looking ahead, the AI landscape is filled with both promise and uncertainty. OpenAI's recent advancements, such as reasoning-based models and task-oriented agents, hint at a future where AI can perform complex tasks independently. However, the ultimate goal of achieving artificial general intelligence (AGI)—a system that can think and reason like a human—remains a contentious topic. Predictions about when AGI will be realised vary widely, with some experts suggesting it could happen soon, while others believe it may take decades or even centuries.
Conclusion
As the AI industry navigates these challenges, the excitement surrounding its potential must be tempered with a realistic understanding of the hurdles ahead. The journey towards more sophisticated AI systems is fraught with complexities, and the path to profitability is anything but clear. The future of AI will depend on how well companies can adapt to these realities while continuing to innovate and push the boundaries of what is possible.