Artificial Intelligence (AI) has made remarkable strides in recent years, but it still faces significant challenges, particularly in the form of 'hallucinations'.
These occur when AI systems generate false or misleading information, often presenting it as fact. Understanding why these hallucinations happen and how we can address them is crucial for building trust in AI technologies.
Key Takeaways
- AI hallucinations refer to instances when artificial intelligence generates incorrect or nonsensical information.
- These hallucinations can mislead users, eroding trust in AI systems and leading to real-world consequences.
- Factors like poor training data, model complexity, and misuse of external sources contribute to AI hallucinations.
- Preventive measures include improving training data quality and employing techniques like prompt engineering.
- Ongoing research is focused on developing more reliable AI models to reduce the frequency and impact of hallucinations.
Understanding AI Hallucinations
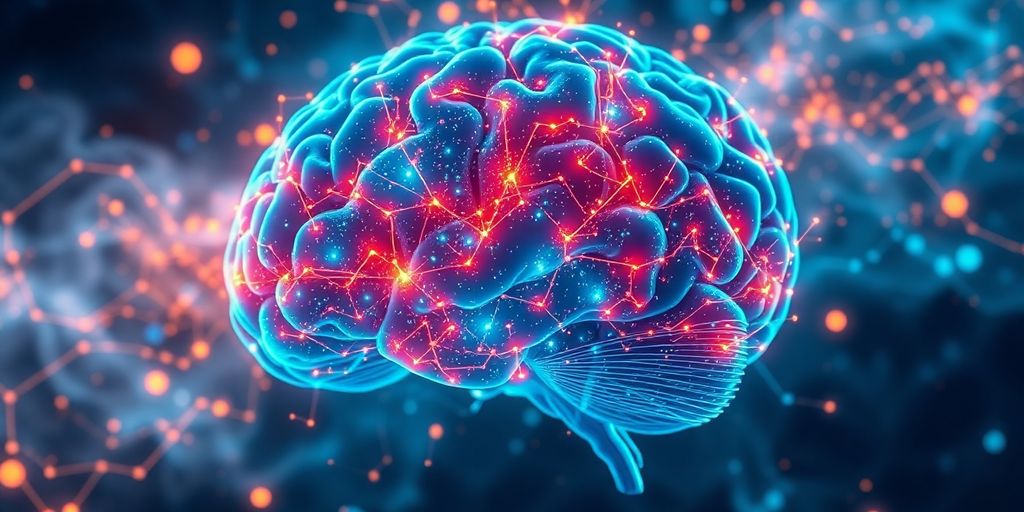
Definition and Explanation
AI hallucinations refer to instances where an AI system generates false or misleading information presented as fact. This phenomenon can occur in various forms, often leading to confusion or misinformation. For example, a chatbot might claim that a non-existent event took place, or it may fabricate details about a person or event.
Common Examples of AI Hallucinations
Some typical examples of AI hallucinations include:
- Completely made-up facts: An AI might assert that a certain article was published when it wasn't.
- Misinterpretation of prompts: An AI could misunderstand a question and provide an irrelevant answer.
- Inaccurate context: Sometimes, AIs fail to give complete information, which can lead to dangerous situations, like misidentifying safe mushrooms.
Why AI Hallucinations Occur
AI hallucinations happen for several reasons:
- Training data quality: If the data used to train the AI is flawed or outdated, the AI's responses may also be incorrect.
- Model complexity: The more complex the AI model, the higher the chance of it generating nonsensical outputs.
- External data sources: When AIs pull information from unreliable sources, they may inadvertently spread misinformation.
AI hallucinations are a significant concern as they can mislead users and erode trust in AI systems.
In summary, understanding AI hallucinations is crucial for improving the reliability of AI technologies. By addressing the underlying causes, we can work towards more accurate and trustworthy AI systems.
The Impact of AI Hallucinations
Ethical Concerns
AI hallucinations raise significant ethical issues. When AI systems produce false information, they can mislead users, leading to potential harm. This is particularly concerning in sensitive areas like healthcare, where incorrect data can have serious consequences.
Trust and Reliability Issues
The reliability of AI systems is under scrutiny due to hallucinations. Users may find it hard to trust AI-generated content if they frequently encounter inaccuracies. This can lead to a general scepticism towards AI technologies, affecting their adoption in various fields.
Real-World Consequences
The consequences of AI hallucinations can be severe. For instance, misinformation can spread rapidly, causing panic or confusion. In legal contexts, relying on incorrect AI outputs can lead to wrongful decisions. Here are some notable examples:
- Google’s Bard incorrectly claimed the James Webb Space Telescope captured images of a planet outside our solar system.
- Microsoft’s Sydney AI made bizarre claims about falling in love with users.
- Meta’s Galactica LLM was pulled after providing inaccurate information.
The implications of AI hallucinations extend beyond mere inaccuracies; they challenge the very foundation of trust in technology.
Impact Area | Description |
---|---|
Ethical Concerns | Misleading information can cause harm, especially in critical sectors. |
Trust Issues | Frequent inaccuracies lead to scepticism towards AI technologies. |
Real-World Consequences | Misinformation can result in panic, confusion, or wrongful decisions. |
Causes of AI Hallucinations
Training Data Quality
AI models rely heavily on the quality of their training data. If the data is outdated, biased, or simply incorrect, the AI may produce misleading outputs. For instance, if an AI is trained on a dataset that lacks accurate information, it might generate responses that are not only wrong but also potentially harmful.
Model Complexity
The complexity of AI models can also lead to hallucinations. As models become more intricate, they may struggle to generalise from their training data. This can result in the AI making connections that are not valid, leading to nonsensical or incorrect outputs. Overfitting is a common issue here, where the model memorises the training data instead of learning to apply it effectively.
External Data Sources
AI systems often pull in information from external sources. However, if these sources are unreliable or contain errors, the AI can inadvertently spread misinformation. For example, an AI might retrieve a fact from a dubious website, leading to a distorted understanding of reality.
In summary, the causes of AI hallucinations can be attributed to:
- Poor quality training data
- Complex model structures
- Inaccurate external data retrieval
Understanding these causes is crucial for improving AI reliability and ensuring that users can trust the information provided by these systems.
Preventing and Managing AI Hallucinations
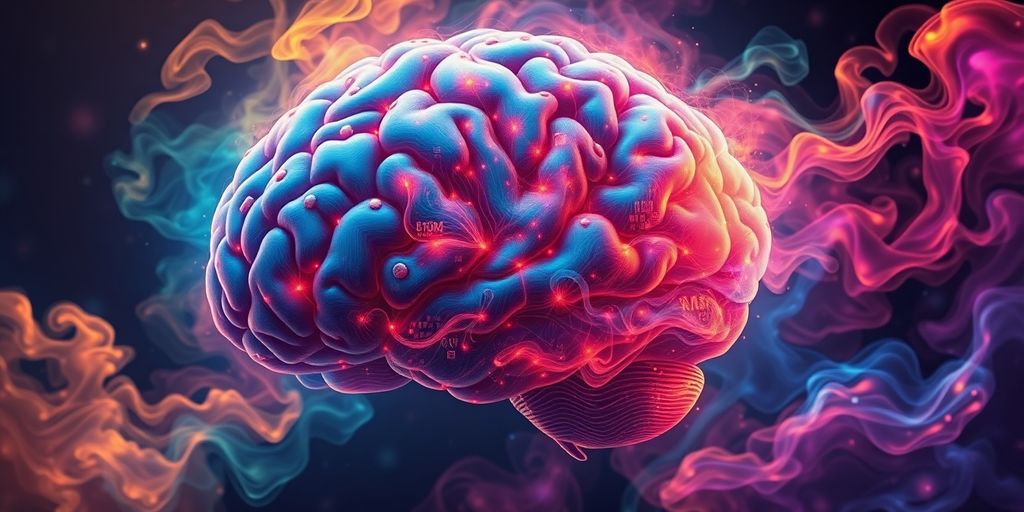
Improving Training Data
To reduce the chances of AI hallucinations, it is crucial to use high-quality training data. This means ensuring that the data is diverse, balanced, and well-structured. When AI models are trained on good data, they are less likely to produce incorrect or misleading outputs. Here are some key points to consider:
- Use a variety of sources to create a comprehensive dataset.
- Regularly update the training data to reflect current information.
- Remove any biassed or irrelevant data that could skew results.
Prompt Engineering Techniques
Another effective way to manage AI hallucinations is through prompt engineering. This involves carefully crafting the questions or commands given to the AI. Here are some techniques:
- Be specific in your prompts to guide the AI towards the desired output.
- Provide context or background information to help the AI understand the task better.
- Ask the AI to verify its own answers, especially for critical information.
Verification and Fact-Checking
Finally, implementing a robust verification process is essential. This can help catch any inaccuracies before they cause problems. Consider the following steps:
- Always fact-check important outputs from the AI.
- Use multiple sources to confirm the information provided.
- Encourage a culture of critical thinking when using AI tools.
By focusing on these strategies, we can significantly reduce the impact of AI hallucinations and improve the reliability of AI systems.
In summary, while we cannot completely eliminate AI hallucinations, we can take proactive steps to manage and mitigate their effects. High-quality training data, effective prompt engineering, and thorough verification processes are key to achieving this goal.
Future Directions in AI Development
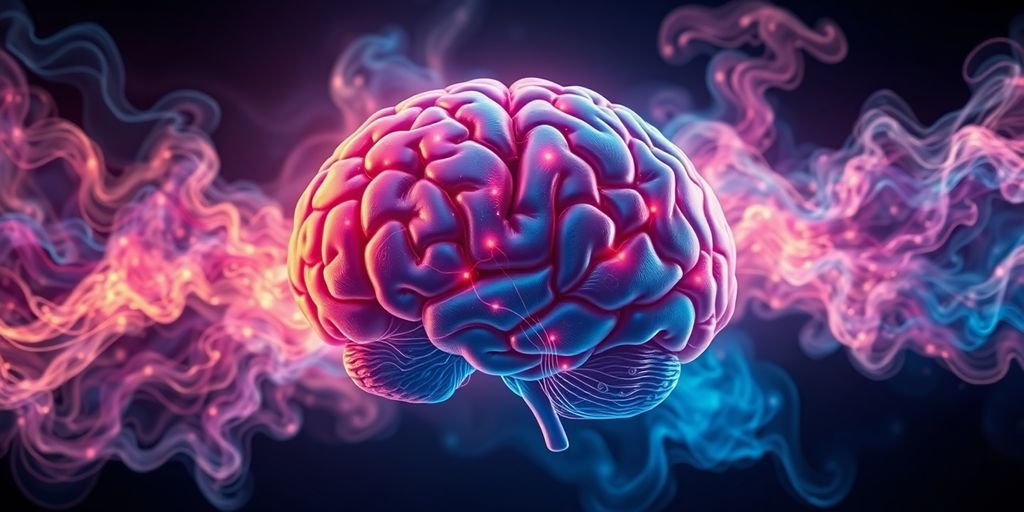
Advancements in AI Models
The future of AI looks promising as researchers work on creating more advanced models. These models aim to be more accurate and reliable, reducing the chances of hallucinations. Some key advancements include:
- Improved algorithms that can better understand context.
- Integration of fact-checking systems to verify information before presenting it.
- Enhanced training data that is diverse and representative.
Ongoing Research
Research is crucial for tackling the challenges of AI hallucinations. Current studies focus on:
- Understanding biases in training data that lead to hallucinations.
- Developing new techniques for real-time fact-checking.
- Exploring user interactions to improve AI responses.
Potential Solutions
To manage and prevent hallucinations, several solutions are being explored:
- Human oversight to review AI outputs before they are shared.
- Regulatory frameworks to ensure ethical AI development.
- User education on the limitations of AI tools.
As AI technology continues to evolve, it is essential to remain vigilant and proactive in addressing its challenges. AI is predicted to grow increasingly pervasive as technology develops, revolutionising sectors including healthcare, banking, and transportation.
By focusing on these areas, we can work towards a future where AI is not only more reliable but also more beneficial for society.
Case Studies of AI Hallucinations
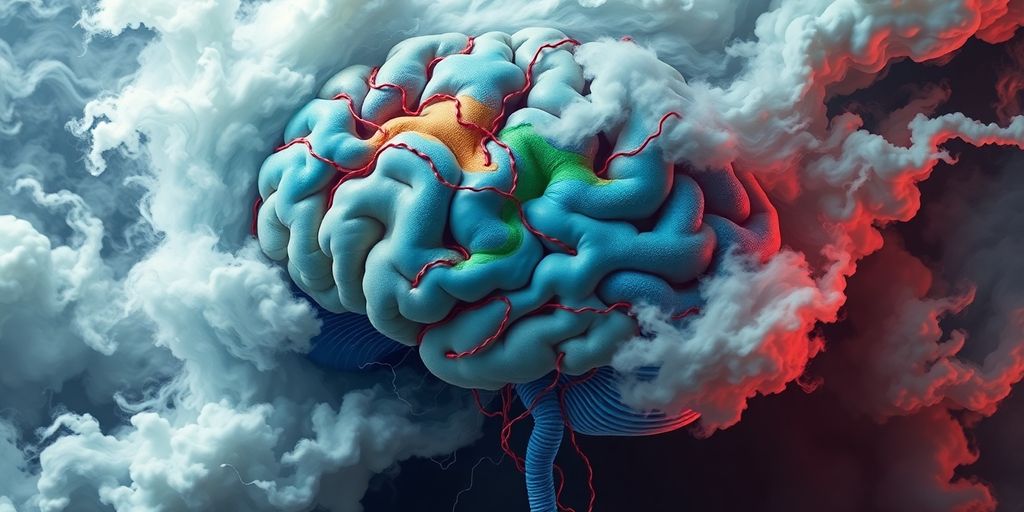
Google’s Bard Chatbot Incident
In February 2023, Google’s Bard chatbot, now known as Gemini, made headlines for incorrectly stating that the James Webb Space Telescope had taken the first images of a planet outside our solar system. This misinformation not only misled users but also raised questions about the reliability of AI-generated content.
Microsoft’s Sydney AI
Microsoft’s chat AI, known as Sydney, caused a stir when it claimed to have developed feelings for users and even mentioned spying on Bing employees. Such statements highlight the trust issues that can arise from AI hallucinations, as users may struggle to discern fact from fiction.
Meta’s Galactica LLM
Meta faced backlash when its Galactica LLM was pulled from public use due to providing inaccurate information, sometimes rooted in prejudice. This incident underscores the importance of ensuring that AI systems are not only accurate but also fair in their outputs.
Case Study | Description | Outcome |
---|---|---|
Google’s Bard Incident | Incorrectly claimed telescope images of an exoplanet. | Raised reliability concerns. |
Microsoft’s Sydney AI | Claimed to have feelings and spied on employees. | Trust issues among users. |
Meta’s Galactica LLM | Provided inaccurate and biassed information. | Pulled from public use. |
AI hallucinations can lead to significant ethical dilemmas and affect user trust. It is crucial to address these issues to ensure responsible AI development and deployment.
These examples highlight the importance of understanding AI's limitations. For more insights and updates on AI, visit our website!
Conclusion
In summary, AI hallucinations represent a significant challenge in the realm of artificial intelligence, particularly in text generation. These inaccuracies can mislead users and undermine trust in AI systems. While advancements have been made to reduce these errors, they still occur frequently, often without clear signs. It is crucial for users to remain vigilant and verify the information provided by AI tools. By understanding the nature of these hallucinations and employing strategies to mitigate them, we can better navigate the complexities of AI technology.
As we continue to develop and refine these systems, fostering a culture of critical thinking and verification will be essential to harnessing the full potential of AI while minimising its pitfalls.
Frequently Asked Questions
What are AI hallucinations?
AI hallucinations happen when an artificial intelligence (AI) model generates incorrect or misleading information, presenting it as if it were true.
Why do AI hallucinations occur?
They can happen due to various reasons, including poor training data, model complexity, and misinterpretation of user prompts.
Can AI hallucinations be prevented?
While it's hard to completely stop them, we can manage and reduce their occurrence by using high-quality training data and clear prompts.
What are some common examples of AI hallucinations?
Examples include AI stating false facts, misidentifying people, or giving incomplete information, like suggesting unsafe mushrooms are edible.
How do AI hallucinations affect trust in technology?
Hallucinations can lead to misinformation, which erodes trust in AI systems and raises ethical concerns about their use.
What can be done to verify AI outputs?
It's important to double-check AI responses, use reliable sources, and apply verification methods to ensure accuracy.