Artificial Intelligence (AI) and Machine Learning (ML) are changing the way clinical trials are designed, conducted, and analysed.
These technologies help researchers make better decisions, recruit suitable participants, and analyse large amounts of data. This article explores how AI and ML can improve clinical trials, the challenges involved, and what the future may hold.
Key Takeaways
AI can enhance the planning and design of clinical trials by predicting outcomes and optimising treatment plans.
Machine learning helps in finding the right participants for trials, reducing the chances of drop-outs.
Data collection and analysis are made easier and faster with AI, allowing for better insights from complex data.
While AI offers many benefits, there are ethical concerns such as privacy and bias that need to be addressed.
The future of clinical trials may see more integration of AI with other technologies, improving efficiency and effectiveness.
The Role of Artificial Intelligence in Clinical Trial Design
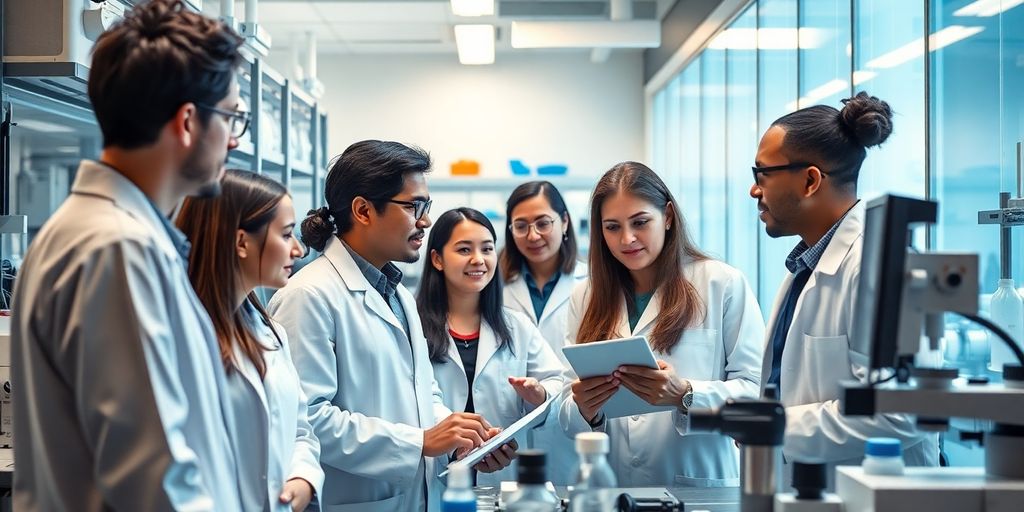
Artificial Intelligence (AI) is changing how clinical trials are designed, making them more efficient and effective. AI helps researchers make better decisions about trial protocols, which can lead to more successful outcomes.
Optimising Dosage and Treatment Regimens
AI can analyse vast amounts of data to determine the best dosages and treatment plans for patients. This includes:
Evaluating previous trial data to find optimal dosages.
Considering patient characteristics to tailor treatments.
Using simulations to predict how different regimens will perform.
Predicting Trial Success with AI
AI-based predictive analytics can forecast the likelihood of a trial's success. For example, algorithms can:
Assess the drug's effectiveness based on historical data.
Identify potential risks early in the trial process.
Adjust trial designs based on real-time data.
Enhancing Protocol Development
AI tools can streamline the development of trial protocols by:
Automating the extraction of eligibility criteria from trial descriptions.
Identifying potential issues in trial designs before they start.
Using past data to inform new trial designs, ensuring they are robust and well-structured.
The integration of AI in clinical trial design not only improves efficiency but also enhances the quality of research outcomes. By leveraging AI, researchers can make informed decisions that lead to better patient care and more successful trials.
In summary, AI is a powerful tool in clinical trial design, helping to optimise treatment regimens, predict outcomes, and enhance protocol development. Its role is becoming increasingly vital as the industry seeks to improve the efficiency and effectiveness of clinical research.
Artificial Intelligence in Participant Recruitment and Management
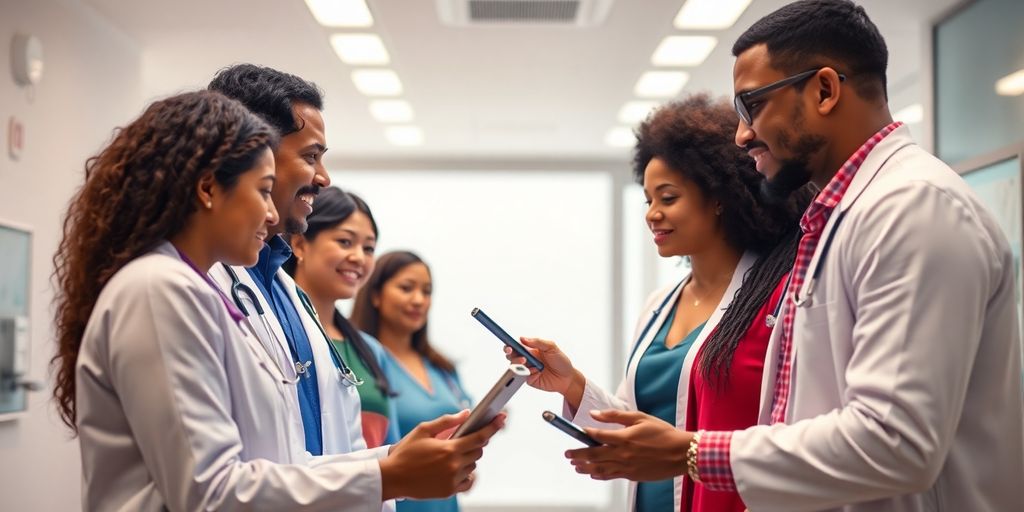
Identifying Ideal Trial Sites
Artificial intelligence can significantly enhance the process of finding the right locations for clinical trials. By analysing various data sources, AI can pinpoint sites that have a higher likelihood of recruiting suitable participants. This targeted approach ensures that trials are conducted in areas where potential participants are more likely to be found, thus improving recruitment efficiency.
Optimising Eligibility Criteria
AI can also help refine the eligibility criteria for trials. By using data mining techniques, researchers can identify the most relevant factors that predict participant success. This means that trials can be designed to include a more diverse range of participants, which is crucial for the generalisability of results. Here are some key benefits of optimising eligibility criteria:
Increased diversity in participant demographics.
Higher recruitment rates due to broader criteria.
Improved trial outcomes by including varied patient profiles.
Reducing Participant Drop-out Rates
One of the major challenges in clinical trials is participant retention. AI can play a vital role in addressing this issue. By analysing past data, AI can predict which participants are at risk of dropping out and allow researchers to intervene early. Some strategies include:
Monitoring participant engagement through regular check-ins.
Providing tailored support based on individual needs.
Utilising chatbots to answer participant queries and concerns.
AI holds promise for optimising recruitment in clinical trials, but its effectiveness requires further validation. Future research should focus on using valid methods to ensure success.
In summary, the integration of artificial intelligence in participant recruitment and management not only streamlines processes but also enhances the overall experience for participants, making clinical trials more effective and inclusive.
Data Collection and Analysis Using Machine Learning
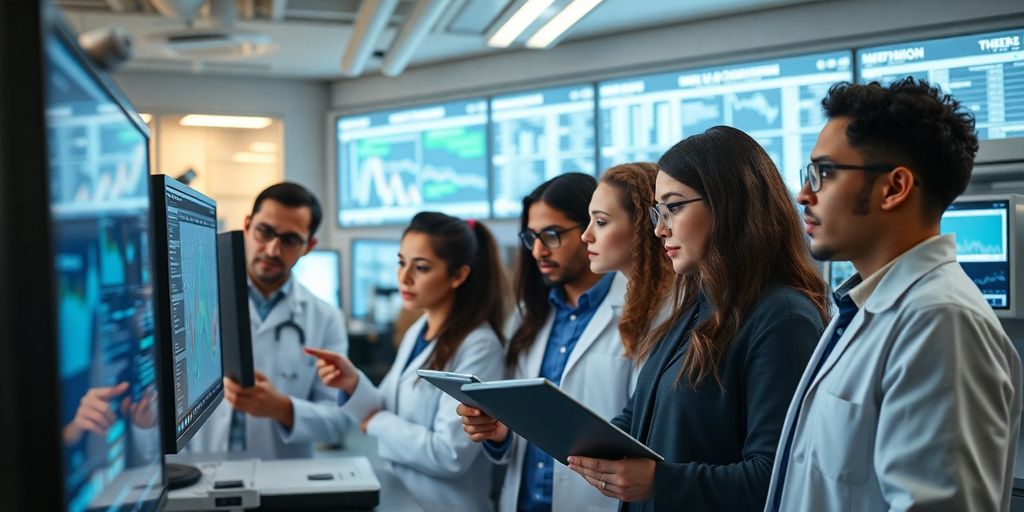
Automating Data Collection Processes
Machine learning (ML) can significantly enhance the data collection process in clinical trials. By automating data entry from various sources, such as wearable devices and electronic health records (EHRs), researchers can reduce human error and save time. This automation can lead to more accurate and timely data, which is crucial for trial success.
Analysing Complex Data Sets
ML techniques are particularly effective in analysing large and complex data sets. They can identify patterns and trends that might be missed by traditional methods. For example, unsupervised learning can help in discovering hidden relationships within the data, which can inform future research directions.
Predicting Trial Outcomes
One of the most promising applications of ML is in predictive analytics. By using historical data from previous trials, ML algorithms can create models that forecast the likelihood of success for new trials. This capability allows researchers to make informed decisions about trial design and resource allocation.
The integration of machine learning in clinical trials not only streamlines processes but also enhances the overall quality of data analysis.
Data Analysis Techniques | Description |
---|---|
Supervised Learning | Uses labelled data to predict outcomes. |
Unsupervised Learning | Identifies patterns in unlabelled data. |
Reinforcement Learning | Learns optimal actions through trial and error. |
In summary, the application of machine learning in data collection and analysis is transforming clinical trials. By automating processes, analysing complex data, and predicting outcomes, ML is paving the way for more efficient and effective research.
Ethical and Practical Challenges of AI in Clinical Trials
Addressing Bias in AI Models
AI models can sometimes be biased, which means they might not treat all groups of people fairly. This can lead to incorrect results that do not represent the entire population. To tackle this issue, researchers should:
Use diverse datasets to train AI models.
Regularly audit AI systems to check for bias.
Involve a variety of stakeholders in the development process.
Ensuring Patient Privacy and Data Security
When using AI in clinical trials, it is crucial to protect patient information. This includes:
Anonymising data to keep identities safe.
Implementing strong security measures to prevent data breaches.
Ensuring that data is only used for its intended purpose.
Overcoming Dependence on AI
While AI can be very helpful, there is a risk that researchers might rely too much on it. This can lead to:
A lack of understanding of the underlying processes.
Reduced critical thinking skills among researchers.
Potential errors if the AI system fails or provides incorrect information.
It is essential to balance the use of AI with human expertise to maintain trust and ensure accurate results.
In summary, while AI has the potential to improve clinical trials, it is important to address these ethical and practical challenges to ensure that it is used responsibly and effectively.
Future Directions for AI in Clinical Trials
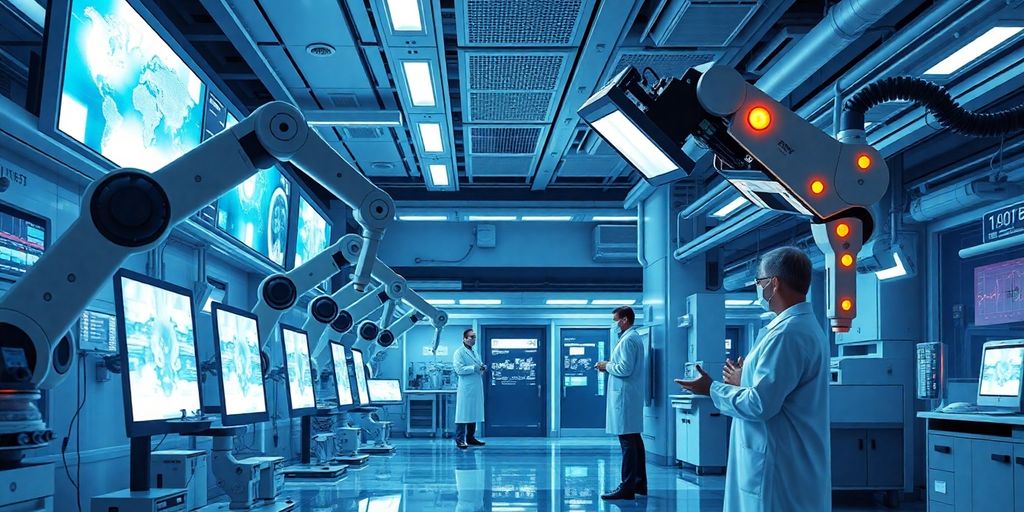
Integrating AI with Emerging Technologies
The future of clinical trials is bright with the integration of AI and other emerging technologies. This combination can lead to:
Enhanced data collection methods, such as wearables and mobile apps.
Improved patient monitoring through real-time data analysis.
Greater collaboration between AI systems and healthcare professionals.
Expanding AI Applications in Clinical Research
AI's role in clinical research is set to grow. Potential applications include:
Personalised treatment plans based on individual patient data.
Advanced predictive models for trial outcomes.
Streamlined processes for patient recruitment and management.
Addressing Regulatory and Compliance Issues
As AI becomes more prevalent, it is crucial to tackle regulatory challenges. Key considerations include:
Ensuring patient privacy and data security.
Developing guidelines for the ethical use of AI in trials.
Creating standards for AI model transparency to build trust.
The integration of AI in clinical trials can revolutionise the way we conduct research, leading to better patient outcomes and more efficient processes.
In summary, the future of AI in clinical trials holds immense potential. By addressing challenges and embracing new technologies, we can enhance the effectiveness and efficiency of clinical research.
Case Studies of AI Implementation in Clinical Trials
Success Stories in AI-Driven Trials
AI has shown remarkable success in various clinical trials, leading to improved outcomes and efficiency. One notable example is the use of digital twins by a start-up called Unlearn. This innovative approach allows researchers to create virtual representations of patients, which can predict how they would have fared in a control group. This method has reduced the number of control patients needed by 20% to 50%, benefiting both researchers and participants.
Lessons Learned from AI Failures
While there are many success stories, there have also been failures in AI implementation. Some key lessons include:
Understanding the limitations of AI models, as they can sometimes produce biassed results.
The importance of data quality; poor data can lead to inaccurate predictions.
Ensuring transparency in AI processes to maintain trust among stakeholders.
Innovative AI Tools and Platforms
Several companies are developing tools that integrate AI into clinical trials. For instance, AutoCriteria helps extract eligibility requirements from trial descriptions, streamlining the recruitment process. Additionally, platforms like ChatTrial allow researchers to query trial databases easily. These tools are transforming how clinical trials are conducted, making them more efficient and effective.
AI is not just a tool; it is a game-changer in clinical trials, offering new ways to enhance patient care and operational efficiency.
Category | 2001–05 | 2011–15 | 2016–20 | 20-year Overall Rise |
---|---|---|---|---|
Endpoints* | 7 | 13 | 22 | 214% |
Procedures* | 110 | 187 | 263 | 139% |
Eligibility Criteria* | 31 | 30 | 30 | -3% |
Countries* | 6 | 9 | 15 | 150% |
Data Points Collected* | 494,236 | 929,203 | 3,453,133 | 599% |
This table illustrates the significant growth in various aspects of clinical trials over the past two decades, highlighting the increasing complexity and data collection efforts. AI is at the forefront of this evolution, paving the way for more effective clinical trials.
In the world of clinical trials, artificial intelligence is making a big difference. By using AI, researchers can speed up processes and improve results. If you want to learn more about how AI is changing healthcare, visit our website for the latest updates and insights!
Conclusion
In summary, the use of Artificial Intelligence (AI) and Machine Learning (ML) in clinical trials shows great promise for enhancing the way we conduct medical research. These technologies can help make trials more efficient, improve patient care, and lead to better outcomes. By analysing large amounts of data, AI can identify patterns that humans might miss, which can help in making informed decisions about treatments.
However, there are still challenges to overcome, such as ensuring the fairness of AI systems and addressing concerns about data privacy. As we continue to explore these technologies, it is crucial to ensure that they are used responsibly and ethically, so that they can truly benefit patients and the healthcare system as a whole.
Frequently Asked Questions
What is the purpose of using Artificial Intelligence in clinical trials?
Artificial Intelligence (AI) helps to make clinical trials better by improving how they are designed, finding the right people to participate, and analysing the data collected.
How does AI help in recruiting participants for trials?
AI can identify the best places to find participants and help set clear rules for who can join the trial, making it easier to get the right people involved.
Can AI predict the success of a clinical trial?
Yes, AI can look at past data and patterns to make predictions about whether a trial is likely to succeed, helping researchers make better decisions.
What are some challenges of using AI in clinical trials?
Some challenges include ensuring that AI models are fair and unbiased, protecting patient privacy, and making sure researchers do not rely too much on AI.
How does AI improve the analysis of clinical trial data?
AI can quickly process large amounts of data, find patterns that humans might miss, and help predict the outcomes of trials more accurately.
What does the future hold for AI in clinical trials?
The future looks bright for AI in clinical trials, with new technologies being developed that could improve how trials are designed, conducted, and analysed.