The convergence of Machine Learning (ML) and the Internet of Things (IoT) is revolutionising how devices operate and interact with users.
Here we delve into the various ways ML enhances IoT, from improving efficiency to transforming user experiences, while also addressing security concerns and future trends. As we explore these areas, it becomes clear that the integration of these technologies is not just a trend but a significant shift that will shape the future of connected devices.
Key Takeaways
Machine Learning helps IoT devices analyse data instantly, making them smarter and more efficient.
Personalised experiences are created through ML, improving how users interact with smart home devices.
Security measures like multi-factor authentication are crucial for protecting IoT systems from threats.
Innovative applications of ML in IoT are emerging, especially in smart cities and healthcare.
Challenges like data volume and compatibility need to be addressed for better integration of ML in IoT.
The Role of Machine Learning in Enhancing IoT Efficiency
Machine learning (ML) is becoming a vital part of the Internet of Things (IoT), helping devices work better and smarter. By using ML, devices can analyse data in real-time, which leads to improved efficiency and smarter decision-making.
Real-Time Data Analysis and Decision Making
With ML, IoT devices can process large amounts of data quickly. This allows them to:
Make decisions instantly based on current information.
Identify patterns and trends that help in understanding user behaviour.
Respond to changes in the environment without human intervention.
Optimising Resource Allocation
ML helps in using resources more effectively. For example:
Devices can learn when to use energy and when to save it.
They can adjust their operations based on demand, reducing waste.
This leads to cost savings and a smaller environmental footprint.
Predictive Maintenance in Industrial IoT
In industries, ML can predict when machines might fail. This is important because:
It helps in scheduling maintenance before problems occur.
Reduces downtime, keeping production running smoothly.
Saves money by avoiding costly repairs and replacements.
The integration of machine learning into IoT is not just about efficiency; it’s about creating systems that can learn and adapt, making our lives easier and more connected.
In summary, machine learning is transforming how IoT devices operate, making them more efficient and capable of meeting user needs effectively. This shift is paving the way for smarter, more responsive technology in our daily lives.
Transforming User Experience Through Machine Learning in IoT
Personalised Smart Home Solutions
Machine learning is revolutionising smart homes by enabling devices to learn from user behaviour. This allows for tailored experiences that adapt to individual preferences. For instance, smart thermostats can adjust temperatures based on past usage patterns, ensuring comfort while saving energy.
Improving Customer Interactions
Businesses are leveraging machine learning to enhance customer service. By analysing data from various sources, companies can predict customer needs and respond proactively. This leads to:
Faster response times
More relevant product recommendations
Improved customer satisfaction
Adaptive Learning in Consumer Devices
Consumer devices are becoming smarter through adaptive learning. For example, smart speakers can recognise different voices and adjust their responses accordingly. This capability not only improves user experience but also fosters a sense of personal connection with technology.
In the world of IoT, machine learning is not just a tool; it’s a game changer that transforms how we interact with our devices, making them more intuitive and responsive to our needs.
Feature | Traditional Devices | Machine Learning Devices |
---|---|---|
User Adaptation | Limited | Continuous |
Data Processing | Centralised | Edge Computing |
Personalisation | Static | Dynamic |
Security and Privacy Concerns in Machine Learning-Driven IoT
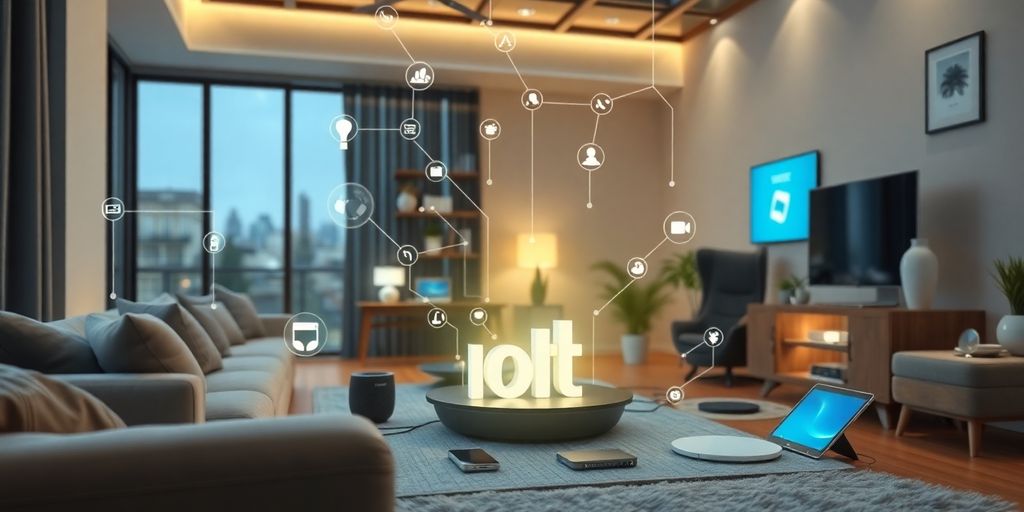
As the integration of machine learning into IoT devices grows, so do the concerns regarding security and privacy. The interconnected nature of these devices makes them vulnerable to various threats. Here are some key areas of concern:
Implementing Multi-Factor Authentication
Multi-factor authentication (MFA) is essential for securing IoT devices. It requires users to provide multiple forms of verification, significantly reducing the risk of unauthorised access.
This method enhances security by ensuring that only authenticated users can access sensitive data.
Regular updates and monitoring of authentication systems are crucial to maintain their effectiveness.
Data Encryption and Secure Storage
Data encryption is vital for protecting sensitive information collected by IoT devices. By encrypting data, organisations can safeguard it from unauthorised access.
Secure storage solutions must be implemented to ensure that personal data remains protected, even if a device is compromised.
Regular audits of data storage practises can help identify potential vulnerabilities.
Addressing Data Drift and System Reliability
Data drift can occur when the data used to train machine learning models changes over time, leading to decreased accuracy. Organisations must continuously monitor and update their models to ensure reliability.
Implementing robust monitoring systems can help detect anomalies and maintain system integrity.
Regular training and validation of models are necessary to adapt to changing data environments.
The rapid advancement of IoT technology necessitates a proactive approach to security and privacy. Organisations must stay ahead of potential threats to protect user data and maintain trust.
By focusing on these areas, businesses can enhance the security and privacy of their machine learning-driven IoT systems, ensuring a safer environment for users and their data.
Innovative Applications of Machine Learning in IoT
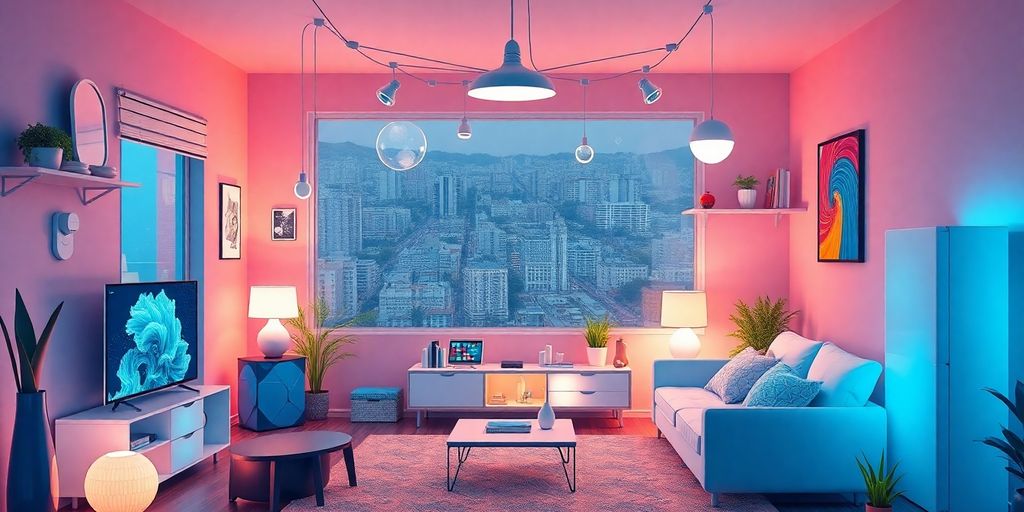
Machine learning is revolutionising the Internet of Things (IoT) by enabling devices to perform tasks that were once thought to be impossible. This technology is paving the way for smarter, more efficient systems.
Smart Cities and Transportation Systems
Traffic Management: Machine learning algorithms analyse real-time traffic data to optimise traffic flow and reduce congestion.
Public Safety: Smart surveillance systems use machine learning to detect unusual activities and alert authorities.
Waste Management: IoT sensors monitor waste levels in bins, allowing for efficient collection routes.
Healthcare Monitoring and Feedback
Wearable Devices: Smartwatches and fitness trackers use machine learning to monitor health metrics and provide personalised feedback.
Remote Patient Monitoring: Devices can alert healthcare providers about critical changes in a patient’s condition.
Predictive Analytics: Machine learning helps predict potential health issues based on historical data.
Environmental Monitoring and Conservation
Wildlife Tracking: IoT devices equipped with machine learning can monitor endangered species and their habitats.
Pollution Control: Sensors can detect air and water quality, providing data for timely interventions.
Resource Management: Machine learning optimises the use of natural resources, ensuring sustainability.
The integration of machine learning in IoT is not just about efficiency; it’s about creating a better future for our communities and the environment.
Challenges and Solutions in Integrating Machine Learning with IoT
Integrating machine learning (ML) with the Internet of Things (IoT) brings numerous benefits, but it also presents several challenges that need to be addressed. Here are some key issues and potential solutions:
Scalability Issues and Standardisation
Compatibility with existing systems can be a major hurdle. Many devices use different frameworks, making it hard to scale ML applications.
To tackle this, organisations should adopt standardised protocols that allow various devices to communicate effectively.
Collaboration with industry partners can help create a unified approach to device integration.
Compatibility with Various Hardware
The diversity of IoT devices means that not all hardware can support advanced ML algorithms. This can lead to inefficiencies.
Developing middleware solutions can help bridge the gap between different devices and platforms, ensuring smoother integration.
Regular updates and maintenance of devices can also enhance compatibility over time.
Overcoming Data Volume Challenges
The sheer amount of data generated by IoT devices can overwhelm systems, making it difficult to process and analyse effectively.
Implementing robust data management strategies is essential. This includes using cloud storage solutions that can scale with demand.
Automated data processing tools can help streamline analytics, allowing organisations to focus on actionable insights rather than data overload.
Addressing these challenges requires a proactive approach, ensuring that organisations can fully leverage the potential of machine learning in their IoT ecosystems.
By understanding and tackling these issues, businesses can enhance their operational efficiency and drive innovation in the rapidly evolving IoT landscape.
Future Trends in Machine Learning and IoT
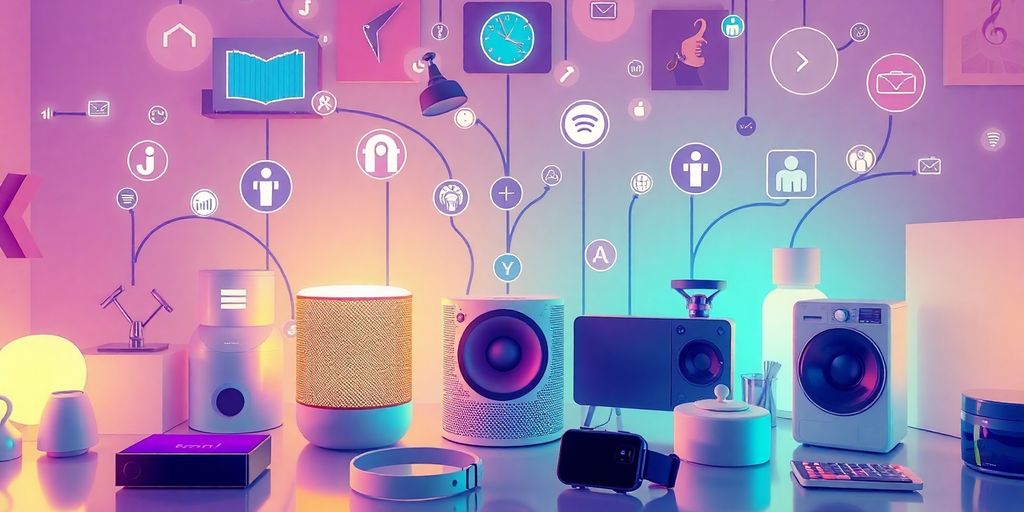
As we look ahead, the integration of machine learning with the internet of things is set to revolutionise how devices operate and interact. Here are some key trends to watch:
Advancements in Edge Computing
Edge computing allows data processing to occur closer to the source, reducing latency and improving response times.
This shift enables devices to operate more independently, enhancing their ability to make real-time decisions.
With more processing power at the edge, devices can handle complex tasks without relying heavily on cloud resources.
Integration of Large Language Models
The use of large language models (LLMs) in IoT devices is becoming more common, enabling smarter interactions.
These models can understand and generate human-like text, improving user interfaces and customer support.
For instance, smart assistants can provide more accurate responses and learn from user interactions over time.
Emerging Use Cases and Opportunities
Smart cities: Enhanced traffic management and energy efficiency through connected devices.
Healthcare: Real-time patient monitoring and personalised treatment plans using data from wearable devices.
Environmental conservation: IoT devices can monitor ecosystems and provide data for conservation efforts.
The future of machine learning in IoT is not just about efficiency; it’s about creating a more connected and intelligent world.
As these trends unfold, businesses and consumers alike will benefit from smarter, more responsive devices that enhance everyday life.
The Impact of Machine Learning on Business and Industry
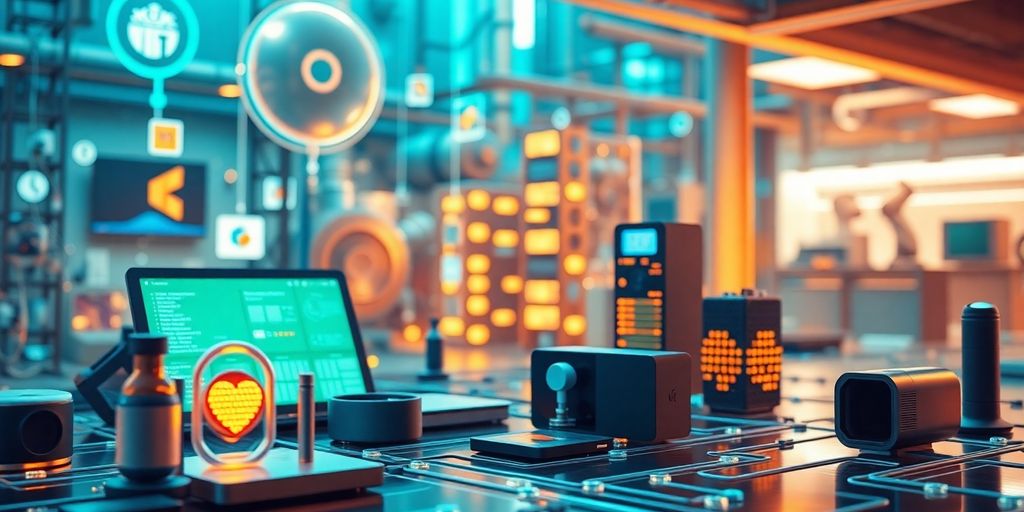
Machine learning (ML) is revolutionising how businesses operate, leading to significant improvements in efficiency and innovation. The integration of ML with IoT is creating a new era of technological integration that is reshaping industries.
Enhancing Operational Efficiency
Real-time data analysis allows companies to make informed decisions quickly.
Predictive maintenance helps in identifying potential equipment failures before they occur, saving costs and reducing downtime.
Automation of routine tasks leads to increased productivity and reduced human error.
Driving Innovation and Competitive Advantage
Businesses can leverage ML to develop smarter products that adapt to user preferences.
Companies that utilise ML can respond to market changes more swiftly, maintaining a competitive edge.
The ability to analyse vast amounts of data enables organisations to uncover new opportunities and trends.
Transforming Business Models and Strategies
Data-Driven Decision Making: Companies are shifting from intuition-based decisions to data-driven strategies.
Personalised Customer Experiences: ML allows for tailored services, enhancing customer satisfaction and loyalty.
New Revenue Streams: Innovative applications of ML can lead to the creation of new products and services.
The future of business lies in the ability to harness data effectively, and machine learning is at the forefront of this transformation.
Aspect | Impact |
---|---|
Operational Efficiency | Increased productivity and reduced costs |
Innovation | New products and services |
Customer Experience | Personalised interactions |
Decision Making | Faster and more accurate insights |
Machine learning is changing how businesses operate, making them smarter and more efficient. By using data to make decisions, companies can improve their services and products. If you want to learn more about how machine learning can benefit your business, visit our website for the latest insights and tools!
Conclusion
In conclusion, the future of the Internet of Things (IoT) is being significantly influenced by machine learning. This powerful combination is changing how devices operate, allowing them to learn from data and make decisions on their own. As these smart devices become more capable, they can better understand our needs and adapt to our preferences. This shift not only enhances our daily lives but also transforms industries by improving efficiency and creating new opportunities. As we move forward, embracing these technologies will be crucial for businesses and individuals alike, paving the way for a more connected and intelligent world.
Frequently Asked Questions
What is machine learning and how does it relate to IoT?
Machine learning is a type of technology that allows computers to learn from data and improve over time. In the context of IoT, it helps devices make smart decisions based on the data they collect.
How can machine learning improve user experience in smart homes?
Machine learning can personalise smart home devices, making them adapt to individual preferences. For example, smart lights can learn when you usually turn them on and adjust automatically.
What are some security risks associated with IoT devices?
IoT devices can be vulnerable to hacking, which may compromise personal data. Using strong passwords and multi-factor authentication can help protect these devices.
How does predictive maintenance work in industrial settings?
Predictive maintenance uses machine learning to monitor equipment and predict when it might fail. This helps businesses fix problems before they cause downtime.
What role does edge computing play in IoT?
Edge computing processes data closer to where it is generated, reducing delays and improving response times. This is important for real-time applications in IoT.
What future trends can we expect in IoT and machine learning?
We can expect advancements in smart cities, healthcare, and environmental monitoring. Machine learning will continue to enhance how devices interact and make decisions.