Artificial Intelligence (AI) is a rapidly evolving field that has become essential in our daily lives.
From smartphones to healthcare, AI systems are designed to learn and adapt, mimicking human thought processes. This article explores how AI learns, the methods it uses, and its impact on various sectors.
Key Takeaways
Artificial Intelligence aims to mimic human thinking and learning.
AI systems improve over time by learning from data and experiences.
Machine Learning is a key part of AI, allowing systems to learn without direct programming.
Neural networks are inspired by the human brain and are crucial for complex tasks.
AI has wide applications, from healthcare to finance, enhancing efficiency and decision-making.
Understanding the Basics of Artificial Intelligence
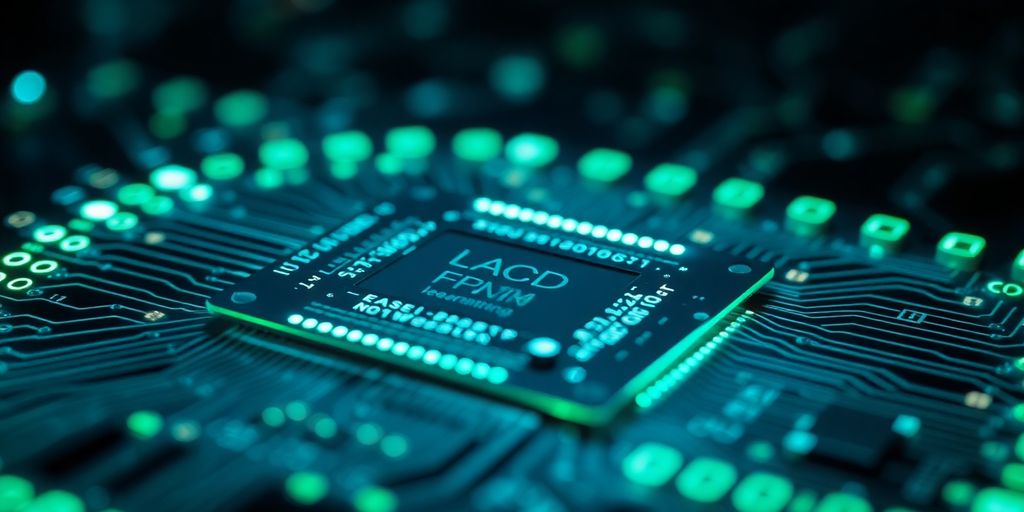
Defining Artificial Intelligence
Artificial intelligence (AI) is a branch of computer science that aims to create machines capable of performing tasks that usually require human intelligence. This includes tasks like reasoning, learning, and problem-solving. AI systems can analyse large amounts of data, identify patterns, and make decisions based on that information.
Historical Background of AI
The term "artificial intelligence" was first used in 1956. Since then, AI has evolved significantly, especially with the rise of big data and advanced algorithms. Early AI research focused on simple problem-solving, but today, AI is integrated into many aspects of our lives, from smart assistants to self-driving cars.
Importance of AI in Modern Society
AI plays a crucial role in various industries, helping to automate tasks and improve efficiency. Here are some key areas where AI is making an impact:
Healthcare: AI assists in diagnosing diseases and personalising treatment plans.
Finance: AI algorithms help in fraud detection and risk assessment.
Transportation: AI powers autonomous vehicles, enhancing safety and efficiency.
AI is not just a technology; it is a transformative force that is reshaping how we live and work.
In summary, understanding the basics of artificial intelligence is essential for anyone looking to navigate the modern world, as it continues to influence various sectors and improve our daily lives.
Area of Impact | Description |
---|---|
Healthcare | AI aids in diagnosis and treatment recommendations. |
Finance | AI enhances fraud detection and financial analysis. |
Transportation | AI enables self-driving technology and traffic management. |
The Core Components of AI Systems
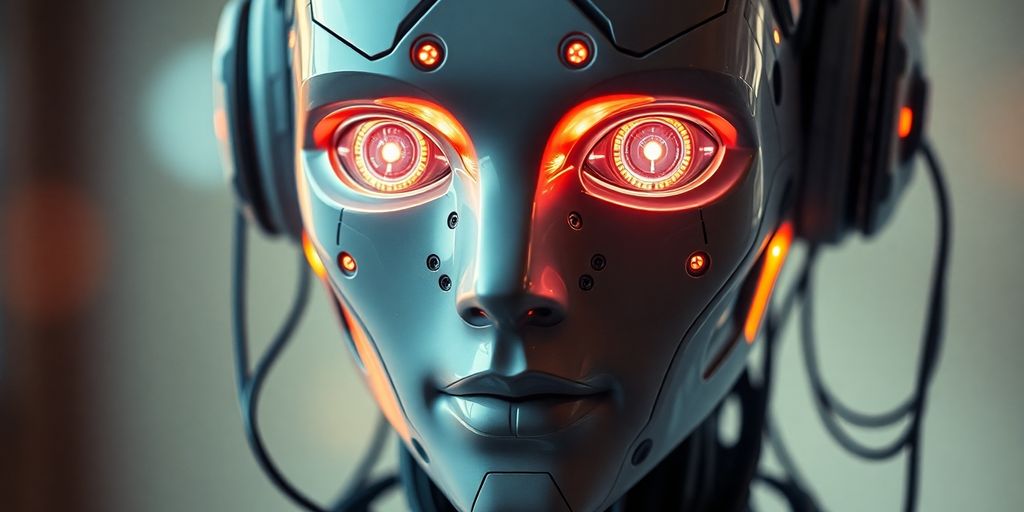
Artificial Intelligence (AI) is built on several key elements that work together to create intelligent systems. These components include algorithms, data, and hardware. Each plays a vital role in ensuring that AI can perform tasks effectively.
Algorithms and Data
Algorithms are the set of rules or instructions that AI systems follow to process data. They help in making decisions based on the information provided. The data used is crucial because it trains the algorithms to recognise patterns and make predictions. Here are some important points about algorithms and data:
Algorithms can be simple or complex, depending on the task.
Data must be accurate and relevant for the AI to learn effectively.
The quality of data directly affects the performance of AI systems.
Machine Learning Models
Machine learning models are a subset of AI that enables systems to learn from data without being explicitly programmed. These models improve over time as they are exposed to more data. Some common types of machine learning models include:
Supervised Learning: The model learns from labelled data.
Unsupervised Learning: The model identifies patterns in unlabelled data.
Reinforcement Learning: The model learns by receiving feedback from its actions.
Neural Networks and Deep Learning
Neural networks are inspired by the human brain and consist of interconnected nodes (or neurons) that process information. Deep learning is a more advanced form of neural networks with multiple layers, allowing for the analysis of complex data. Key features include:
Ability to handle large amounts of data.
Improved accuracy in tasks like image and speech recognition.
Continuous learning from new data inputs.
In summary, the components of an AI system work together to create intelligent solutions that can adapt and improve over time. Understanding these components is essential for grasping how AI functions in various applications.
How Machine Learning Enables AI
The Concept of Machine Learning
Machine learning is a key part of artificial intelligence (AI). It allows computers to learn from data without being told exactly what to do. This means that instead of following strict instructions, machines can improve their performance by finding patterns in the information they receive. For example, a machine can learn to recognise different animals in pictures by looking at many examples.
Types of Machine Learning Algorithms
There are several types of machine learning algorithms that help AI systems learn:
Supervised Learning: This is where the machine learns from labelled data, meaning it knows the correct answer.
Unsupervised Learning: Here, the machine looks for patterns in data without knowing the answers.
Reinforcement Learning: In this type, the machine learns by trying things out and getting feedback on its actions.
Applications of Machine Learning
Machine learning is used in many areas, including:
Healthcare: For diagnosing diseases based on patient data.
Finance: To detect fraud by analysing transaction patterns.
Entertainment: For recommending movies or music based on user preferences.
Machine learning is not just about making predictions; it’s about adapting to new information and improving over time.
In summary, machine learning is a powerful tool that enables AI to learn from experience, making it essential for developing intelligent systems that can perform complex tasks effectively.
The AI Learning Process
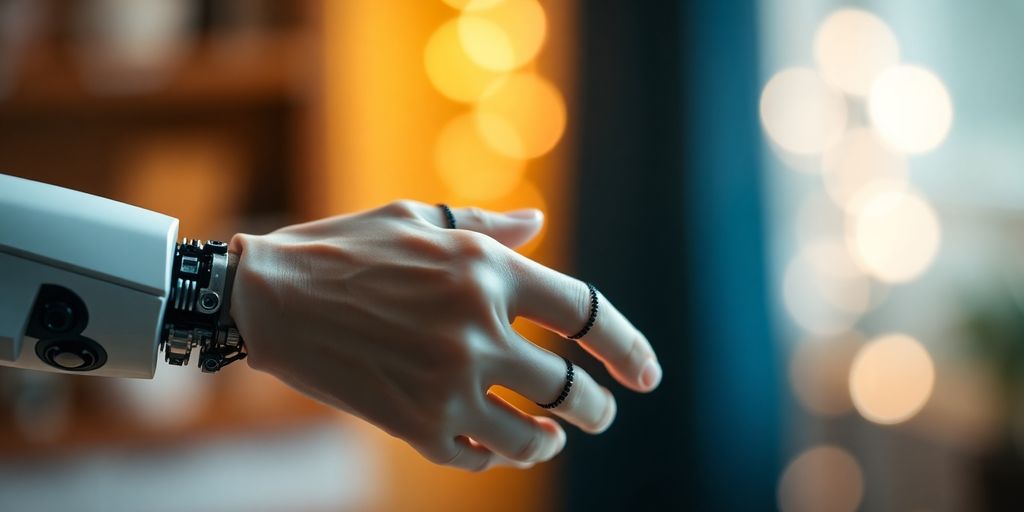
Artificial Intelligence (AI) learns through a series of steps that help it understand and adapt to new information. This process is similar to how humans learn from their experiences. Here are the main stages involved in AI learning:
Data Collection and Preparation
Gathering Data: The first step is to collect relevant data, which serves as the foundation for the AI model. This data can come from various sources, such as sensors, databases, or user inputs.
Cleaning Data: Once collected, the data must be cleaned and organised to ensure it is suitable for training. This involves removing errors and inconsistencies.
Formatting Data: The data is then formatted into a structure that the AI can understand, making it ready for analysis.
Model Training and Validation
Choosing a Model: After preparing the data, the next step is to select an appropriate machine learning model. This choice depends on the type of problem being solved and the nature of the data.
Training the Model: The model is trained using the prepared data. During this phase, the AI learns to identify patterns and make predictions based on the input data.
Validating the Model: Once training is complete, the model is tested with new data to check its accuracy. This helps to ensure that the model can generalise its learning to unseen data.
Continuous Learning and Adaptation
Feedback Loop: AI systems are designed to learn continuously. They can adapt to new data and improve their performance over time.
Monitoring Performance: Regular monitoring is essential to ensure the AI remains effective. Adjustments may be made based on its performance in real-world applications.
Updating Models: As new data becomes available, models can be updated to reflect changes and improve accuracy.
The AI learning process is crucial for developing systems that can operate effectively in dynamic environments, allowing them to make informed decisions and predictions.
In summary, the AI learning process involves collecting and preparing data, training and validating models, and continuously adapting to new information. This structured approach enables AI to function effectively across various applications, from healthcare to finance, showcasing its versatility and importance in modern society.
Applications and Implications of AI Learning
AI learning has significant implications across various sectors, including healthcare, finance, and education. As AI continues to evolve, it is essential to consider the ethical aspects and potential challenges that may arise from its widespread use.
Applications and Implications of AI Learning
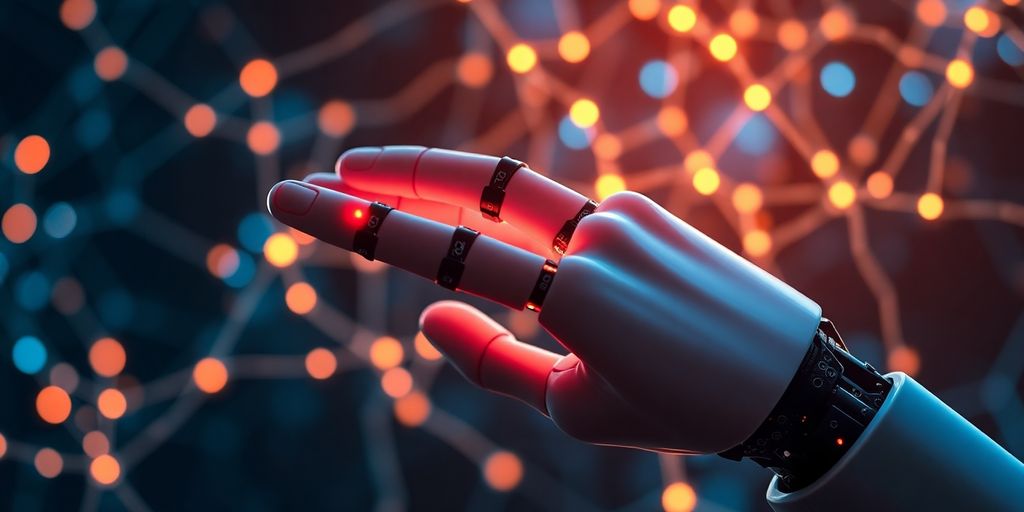
AI in Healthcare
Artificial Intelligence is making a significant impact in the healthcare sector. AI technologies can analyse vast amounts of medical data, helping doctors make better decisions. Some key applications include:
Predictive analytics for patient outcomes.
Personalised medicine tailored to individual needs.
Robotic surgery that enhances precision.
AI in Autonomous Systems
AI is also crucial in developing autonomous systems, such as self-driving cars and drones. These systems rely on AI to:
Process real-time data from their surroundings.
Make decisions based on that data.
Learn from experiences to improve performance over time.
Ethical Considerations in AI Learning
As AI continues to evolve, ethical concerns arise. It is essential to address these issues to ensure responsible use of AI. Some considerations include:
Bias in AI algorithms, which can lead to unfair outcomes.
Privacy concerns regarding data collection and usage.
The impact on jobs, as automation may replace certain roles.
AI has the potential to transform industries, but it is crucial to navigate its implications carefully to ensure it benefits society as a whole.
Application Area | Key Benefits |
---|---|
Healthcare | Improved diagnostics and treatment |
Autonomous Systems | Enhanced safety and efficiency |
Education | Personalised learning experiences |
Challenges and Future Directions in AI Learning
Overcoming Data Limitations
Data is the backbone of machine learning. The quality and completeness of data are vital for training effective AI models. Here are some challenges related to data:
Data Quality: Poor quality data can lead to inaccurate predictions.
Data Availability: Sometimes, there isn't enough data to train models effectively.
Data Bias: If the data is biassed, the AI will also be biassed, leading to unfair outcomes.
Improving Model Accuracy
As AI systems become more complex, ensuring their accuracy is crucial. Some strategies to enhance model accuracy include:
Regular Updates: Continuously updating models with new data helps maintain accuracy.
Cross-Validation: Using different data sets to test models can improve reliability.
Ensemble Methods: Combining multiple models can lead to better performance.
Future Trends in AI Research
The future of AI is bright, with many exciting directions to explore. Some potential trends include:
Reinforcement Learning: This method allows AI to learn from its actions, improving its decision-making abilities. It is particularly important in areas like gaming and robotics, where it can adapt based on feedback.
Human-AI Collaboration: As AI systems become more integrated into daily life, the focus will shift to how humans and AI can work together effectively.
Ethical AI: There will be a growing emphasis on ensuring AI systems are fair and transparent, addressing concerns about bias and accountability.
The journey of AI learning is filled with challenges, but with innovation and collaboration, the future holds great promise for advancements that can benefit society as a whole.
Artificial Intelligence (AI) is facing many challenges as it grows. From understanding complex data to ensuring ethical use, the road ahead is not easy. However, these hurdles also present exciting opportunities for innovation and improvement. If you're curious about the latest developments in AI and want to learn more, visit our website for insightful articles and updates!
Conclusion
In summary, artificial intelligence is a powerful tool that learns and improves over time, much like humans do. By analysing large sets of data, AI can adapt to new situations and make decisions without needing specific instructions. This ability to learn is what makes AI so valuable across various fields, from healthcare to finance.
As technology continues to advance, AI will play an even bigger role in our lives, helping us solve problems and automate tasks more effectively. Understanding how AI learns is essential for harnessing its full potential and ensuring it benefits society.
Frequently Asked Questions
What is artificial intelligence (AI)?
Artificial Intelligence (AI) is a branch of computer science focused on creating machines that can perform tasks that usually require human intelligence, such as understanding language, recognising patterns, and making decisions.
How does AI learn from data?
AI learns by analysing large amounts of data using algorithms, which are sets of rules that help the machine recognise patterns and make predictions based on that data.
What are the different types of machine learning?
There are three main types of machine learning: supervised learning, where the model is trained on labelled data; unsupervised learning, where the model finds patterns in unlabeled data; and reinforcement learning, where the model learns by receiving rewards or penalties.
Why is AI important in today’s world?
AI is important because it helps automate tasks, improves efficiency, and can solve complex problems in various fields like healthcare, finance, and transportation, making our lives easier.
What are some common applications of AI?
Common applications of AI include virtual assistants like Siri and Alexa, recommendation systems on shopping websites, self-driving cars, and medical diagnosis tools.
What challenges does AI face in learning?
AI faces several challenges in learning, such as the need for large amounts of quality data, the risk of bias in algorithms, and the difficulty of ensuring that AI systems make ethical decisions.